Decoding the Role of Wafer Semiconductor Technology in Modern Electronics
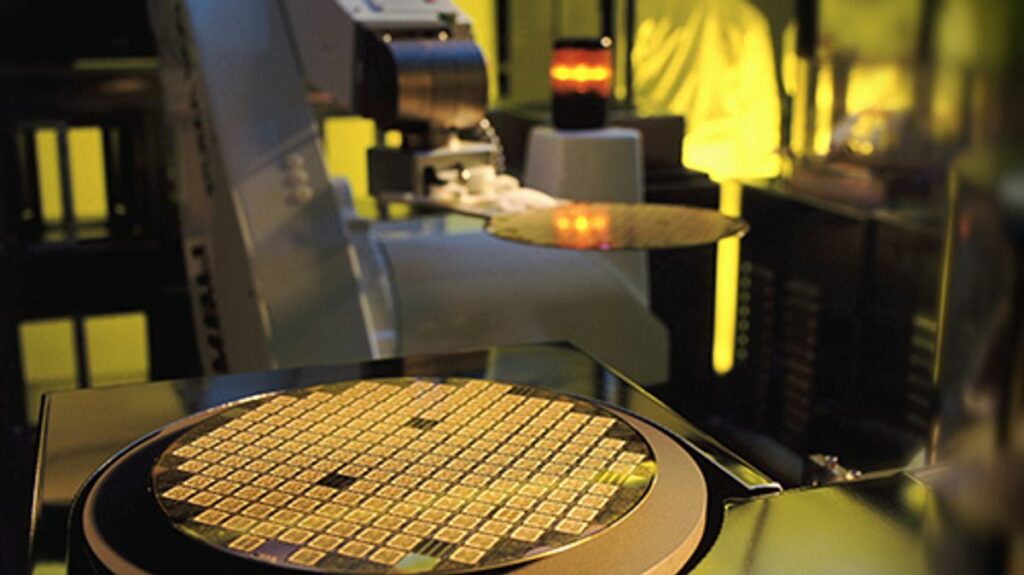
Semiconductor technology has become the backbone of modern electronics, with wafer semiconductor technology playing a pivotal role. The intricate process of manufacturing semiconductor chips involves numerous steps where precision is paramount. As the demand for smaller, faster, and more efficient electronics grows, the technology behind wafer semiconductors continues to evolve, addressing the challenges of defect detection and adapting to the needs of various industries. This article delves into the evolution, challenges, and future perspectives of wafer semiconductor technology, highlighting its significance in the ever-advancing realm of modern electronics.
Key Takeaways
- Wafer semiconductor technology is crucial for the production of high-end chips, with automated defect detection becoming vital due to the limitations of manual inspection.
- Intelligent manufacturing and advances in electronic information technology have transformed the semiconductor process, necessitating stringent control and precision.
- Deep learning and emerging technologies are enhancing the accuracy and efficiency of semiconductor defect detection, overcoming the subjectivity of traditional methods.
- Semiconductor technology integration is vital across industries such as electric vehicles, consumer electronics, telecom, and defense, driving innovation and efficiency.
- Future advancements in semiconductor inspection are expected to leverage AI and novel network structures, balancing the need for confidentiality with research progress.
The Evolution of Wafer Semiconductor Technology
From Manual Inspection to Automated Defect Detection
The transition from manual inspection to automated defect detection marks a significant evolution in the semiconductor manufacturing industry. Automated inspection has become a vital part of quality control during semiconductor wafer production, addressing the inefficiencies and inaccuracies of traditional manual methods.
Wafer defect inspection is critical to ensuring the high standards required in semiconductor manufacturing. The shift to automation was driven by the need for higher efficiency, accuracy, and consistency, which manual visual inspection could not provide. Machine vision technology, an early form of automatic inspection, brought advantages such as high efficiency, reliability, and objectivity.
Recent advancements have seen the integration of industrial big data and artificial intelligence technologies into inspection processes. Deep learning-based methods are now being explored to overcome the limitations of previous systems, offering real-time defect detection and classification. This approach not only enhances the detection of various defect types and sizes but also contributes to material waste reduction and production cost savings.
The Impact of Intelligent Manufacturing on Semiconductor Processes
The adoption and integration of AI applications have emerged as a transformative force in the dynamic landscape of semiconductor manufacturing. Intelligent manufacturing has revolutionized the way semiconductor chips are produced, ensuring a higher degree of precision and control throughout the complex technology chain. From the initial input of raw materials to the final stages of chip packaging, every process is meticulously monitored and optimized.
Traditional manual visual inspection methods, with their high workforce costs and intense subjectivity, are being replaced by automated systems. These systems leverage advanced algorithms and machine learning techniques to enhance defect detection and quality assurance. The shift towards intelligent manufacturing not only improves the quality of semiconductor chips but also significantly reduces the margin for error.
The impact of intelligent manufacturing extends beyond the production floor. It is anticipated to create a ripple effect in downstream industries, leading to increased job prospects in sectors such as automotive, electronics, telecom, and others that heavily rely on semiconductors. By 2026, the semiconductor industry in India alone is expected to generate over 300,000 jobs, covering a wide range of roles from testing and engineering to software development and operations.
Advancements in Chip Quality Inspection Techniques
The relentless pursuit of perfection in semiconductor manufacturing has led to significant improvements in chip quality inspection techniques. It encompasses a variety of methods designed to detect defects and irregularities in semiconductor wafers and devices. With the advent of intelligent manufacturing, the need for more sophisticated inspection methods has become apparent.
Traditional inspection methods, reliant on manual visual checks, are being rapidly outmoded by automated systems. These systems leverage advanced algorithms and machine learning to enhance accuracy and efficiency. For instance, deep learning models can automatically learn and identify various defects through extensive data training, which is a leap forward in both the precision and adaptability of defect detection.
The table below outlines the comparison between traditional and modern inspection methods:
Aspect | Traditional Inspection | Modern Inspection |
---|---|---|
Efficiency | Low | High |
Accuracy | Moderate | Very High |
Cost | High | Reduced |
Labor Intensity | High | Low |
Standardization | Inconsistent | Uniform |
These advancements not only streamline the inspection process but also ensure that the stringent quality demands of high-end chip production are met. As technology evolves, we can anticipate further enhancements in inspection techniques, with deep learning poised to take a central role.
Semiconductor Defect Detection: Challenges and Innovations
The Limitations of Traditional Visual Inspection Methods
Traditional visual inspection methods have long been the cornerstone of quality control in semiconductor manufacturing. However, as the complexity of semiconductor devices has increased, these methods have struggled to keep pace. The main drawbacks of manual visual inspection include low efficiency, low accuracy, and high labor intensity.
Moreover, manual inspections are plagued by inconsistent standards, which can lead to variability in product quality. The high cost associated with skilled labor for manual inspections also adds a significant burden to production expenses. These limitations have prompted the industry to seek more advanced solutions.
The following points summarize the key limitations of traditional visual inspection methods:
- Inconsistent quality standards leading to variable product quality
- Low efficiency, resulting in slower production times
- High labor intensity, requiring significant manpower
- Low accuracy, with a greater likelihood of missing defects
- High costs due to the need for skilled inspectors
Emerging Technologies in Defect Detection and Machine Learning
The advent of machine learning has revolutionized the field of semiconductor defect detection. Powered by neural network models, these emerging technologies are capable of identifying and classifying wafer defects with unprecedented precision. By analyzing vast datasets, machine learning algorithms can discern intricate defect patterns, leading to more accurate and efficient inspections.
One notable advancement is the development of deep learning-based systems, such as the flow-based learning system proposed by Yu and Lu. This system utilizes a joint local and non-local linear discriminant analysis (JLNDA) to extract discriminative features of defect patterns, enhancing the detection process. The application of convolutional neural networks (CNNs) further exemplifies the potential of machine learning in this domain, as they can learn from large amounts of data to automatically recognize various defects.
Despite these innovations, the semiconductor industry faces challenges in adopting these technologies widely. The vital confidentiality of semiconductor data and the complexity of chip environments pose significant hurdles. Nonetheless, the continuous improvement of machine learning models promises to bring higher efficiency and accuracy to semiconductor foundries, supporting the industry’s rapid development.
The Role of Deep Learning in Enhancing Inspection Accuracy
Deep learning has revolutionized the way we approach wafer defect detection, offering a significant leap in accuracy and efficiency. These models excel in environments that are complex and varied, adapting to different chip types with ease. The ability to process and learn from vast datasets allows for the automatic identification of defects, which is crucial for maintaining the high standards required in semiconductor manufacturing.
The integration of deep learning in inspection processes is not without its challenges. The need for confidentiality in semiconductor data, for instance, poses a barrier to research and development in this field. Despite this, the potential benefits are too significant to ignore, with deep learning poised to become even more influential in the future of chip quality inspection.
Recent studies highlight the advancements in this domain. For instance, researchers have developed systems that combine cluster analysis with deep learning, enhancing the generalization capability of defect detection. The table below summarizes the performance of a novel deep learning algorithm, DSCU-Net, in wafer quality inspection:
Metric | Performance (%) |
---|---|
IOU | 72.53 |
Dice | 81.96 |
Accuracy | 87.67 |
As we continue to refine these technologies, the semiconductor industry stands to gain from higher yields and reduced costs, all while pushing the boundaries of what’s possible in electronic device fabrication.
Integrating Semiconductors in Diverse Industries
Semiconductors in Electric Vehicles and Renewable Energy
The integration of semiconductors into the realm of electric vehicles (EVs) and renewable energy sources is a testament to their versatility and critical role in modern technology. Semiconductors are indispensable for green technologies like solar panels and wind turbines, which are essential for the renewable energy transition. In electric vehicles, semiconductors are at the heart of innovation, driving advancements in safety, electrification, communication, and networking.
In India, the surge in EV production is indicative of a broader trend where semiconductors are becoming increasingly vital. This trend is not only transforming the semiconductor industry but also fostering job creation and enhancing chip design capabilities. The use of high-performance compute chips and power management chips is pivotal for the efficient operation of EVs, as well as in other sectors such as telecom and defense.
The following table highlights the projected rise in semiconductor use across various applications in electric vehicles:
Application Area | Importance of Semiconductors |
---|---|
Safety Systems | Crucial for reliable operation |
Electrification | Key to vehicle power management |
Communication | Essential for connectivity |
Networking | Integral for data exchange |
The Influence of Semiconductors on Consumer Electronics and Telecom
Semiconductors have become the cornerstone of modern consumer electronics and telecommunications, driving innovation and enabling the rapid advancement of these industries. The telecommunications sector is increasingly becoming characterized by fixed operator CapEx and increasing user demands, a dynamic that’s not only challenging but also indicative of the critical role semiconductors play in meeting these demands.
In the realm of consumer electronics, semiconductors are integral to the functionality and performance of a wide array of devices. From smartphones to smart home systems, the quality and capabilities of these chips determine the efficiency, connectivity, and user experience. The following table highlights the impact of semiconductor innovation on key consumer electronics categories:
Category | Impact of Semiconductor Innovation |
---|---|
Smartphones | Enhanced processing power and battery life |
Smart TVs | Improved display technologies and interactivity |
Wearables | Advanced sensors and longer device endurance |
Gaming Consoles | Superior graphics and faster load times |
As the demand for high-performance compute chips and power management chips continues to rise, sectors such as electric vehicles (EV), telecom, defense, and consumer electronics are poised for transformation. This demand is not only shaping the semiconductor industry but also the job market, with a projected increase in roles related to testing, engineering, software development, and operations.
Strengthening Chip Design for Defense and Aerospace Applications
The defense and aerospace sectors demand the highest standards in chip design, requiring components that can withstand extreme conditions and offer unparalleled reliability. High-performance compute chips with 28 nm technology are at the forefront of meeting these rigorous requirements, providing the necessary power management for critical applications.
In the realm of defense and aerospace, the integration of advanced semiconductor technology is not just about performance; it’s also about ensuring the security and integrity of the systems. The following table highlights the key attributes that semiconductor chips must possess to be suitable for these industries:
Attribute | Importance |
---|---|
Reliability | Essential for mission-critical operations |
Durability | Must endure harsh environmental conditions |
Security | High-level protection against tampering and cyber threats |
Performance | Capable of processing complex computations quickly |
As the demand for sophisticated electronics in defense and aerospace continues to grow, the semiconductor industry is responding with chips that are not only powerful but also tailored to the unique needs of these applications. This involves a meticulous manufacturing process where every step, from raw material input to final chip packaging, is executed with precision and under strict control to eliminate defects and ensure the highest quality.
The Science Behind Silicon Chip Fabrication
Understanding the Simple Chemistry of Metallization and Etching
The process of metallization in semiconductor fabrication involves depositing a thin metal layer onto the silicon wafer. Traditionally, this is achieved through complex methods like sputtering, which require expensive vacuum equipment. However, innovative approaches have emerged, utilizing simple chemical reactions such as the silver mirror reaction. This reaction, familiar from high school experiments, involves Tollen’s reagent—a mixture of sodium hydroxide, ammonia, and silver nitrate—to create a reflective coating on glass, which can also be adapted for chip metallization.
Etching, on the other hand, is the process of removing material to create the intricate patterns necessary for circuitry. The search for an effective yet accessible etchant led to the use of household items like white vinegar combined with 12% hydrogen peroxide. This mixture proved successful in etching away unwanted silver, although adhesion issues arose, with the silver layer preferring to stick to the etchant rather than the chip. The solution? Replacing the photoresist’s protective film with Teflon thread-sealing tape, which allowed for the entire plating to etching process to function, yielding conductive traces with fine resolution.
While these methods may not match the durability and precision of industrial processes, they offer a fascinating glimpse into the potential for DIY semiconductor projects. The journey from using a homemade tube furnace for oxide layers to refining photolithography techniques underscores the ingenuity and resourcefulness of the maker community in demystifying the complex chemistry behind chip fabrication.
DIY Semiconductor Projects: Bridging the Gap for Hobbyists
The journey from concept to creation in the realm of semiconductors has traditionally been a path trodden by industry professionals. However, the rise of DIY semiconductor projects is empowering hobbyists to delve into the intricacies of chip fabrication. Initiatives like Maker Pro offer a platform for enthusiasts to share designs and collaborate, echoing the sentiment of ‘Your Electronics Projects, From Concept to Creation.’
For those eager to start their own semiconductor fabrication, a DIY tube furnace is an essential piece of equipment. As detailed by Dan Maloney, this tool is a cornerstone for anyone serious about creating semiconductors at home. The process involves simple chemistry to metallize and etch silicon chips, a task that has been demystified by projects such as [ProjectsInFlight].
The community of makers is growing, with individuals like [ProjectsInFlight] and [Sam Zeloof] leading the charge in home semiconductor fabrication. They demonstrate that with the right tools, materials, and safety precautions—such as understanding the risks associated with hydrofluoric acid—ambitious projects are within reach. Here’s a glimpse into the progress and tools used by these pioneers:
- Tube furnace setup
- Metallization and etching techniques
- Safety measures for handling dangerous chemicals
As the DIY semiconductor scene evolves, we can expect to see more sophisticated methods and a wider range of tools available for hobbyists. This not only bridges the gap between professional and amateur chipmakers but also fosters a culture of innovation and shared knowledge.
Optimizing Silicon Chip Production for Efficiency and Scalability
The relentless pursuit of efficiency and scalability in silicon chip production is a cornerstone of the semiconductor industry. Optimization efforts focus on enhancing throughput and yield while minimizing costs and resource consumption. This is achieved through a combination of advanced process control, automation, and continuous improvement methodologies.
Key strategies for optimization include:
- Streamlining the technology chain to reduce complexity and potential points of failure.
- Implementing intelligent manufacturing systems that adapt to changes in real-time.
- Upgrading to equipment that supports finer geometries and higher precision.
- Adopting energy-efficient practices to lower the environmental impact.
The table below illustrates the impact of optimization on production efficiency:
Metric | Before Optimization | After Optimization |
---|---|---|
Throughput (units/day) | 10 million | 15 million |
Defect Rate (%) | 2.0 | 1.5 |
Energy Consumption (MWh) | 50 | 45 |
By focusing on these areas, manufacturers can ensure that their production lines are not only more efficient but also ready to scale up to meet the demands of an ever-growing market for semiconductor devices.
Future Perspectives on Wafer Defect Detection Technology
Proposing Novel Network Structures for Enhanced Detection
The relentless pursuit of perfection in wafer defect detection has led to the proposal of innovative neural network structures. One such advancement is the DSCU-Net, a network model that boasts a complex and dense hopping connection. This structure is designed to significantly enhance the fusion of information between the encoder and decoder, thereby improving feature extraction and defect reconstruction capabilities.
The DSCU-Net, which stands for Dense Skip-Connection U-Net, optimizes the traditional U-Net network by introducing numerous skip connections. These connections facilitate a profound fusion of high-level semantics with low-level details, which is crucial for accurate defect detection. The table below summarizes the key contributions of the DSCU-Net model:
Contribution Point | Description |
---|---|
Network Model | DSCU-Net with dense hopping connections |
Feature Extraction | Enhanced multi-information fusion between encoder and decoder |
Defect Reconstruction | Improved potency in feature extraction and defect detection |
Tested on actual semiconductor production data, DSCU-Net has shown promising results in improving wafer defect detection performance. However, the challenge remains to extend these capabilities to detect unknown defects, which are currently less researched due to the confidentiality of semiconductor data and other constraints.
Balancing Confidentiality with the Need for Advanced Research
In the pursuit of advancing wafer defect detection technology, researchers are often faced with a delicate balance between confidentiality and the open sharing of data. The integrity of intellectual property and sensitive information must be upheld, even as the scientific community pushes for collaborative efforts to enhance research outcomes.
The following points outline the key considerations in this balance:
- Ensuring that proprietary data remains secure while allowing for enough transparency to foster innovation.
- Developing standardized protocols for data sharing that protect confidential information without stifling research.
- Encouraging partnerships between academia and industry to facilitate a controlled exchange of knowledge.
Ultimately, the goal is to create an environment where confidentiality does not become a barrier to progress, but rather a well-managed aspect of the research ecosystem.
Anticipating the Role of AI in Future Semiconductor Inspections
The semiconductor industry is on the cusp of a transformative era with the integration of Artificial Intelligence (AI) in inspection processes. AI’s capability to automatically learn and identify defects through extensive data training is revolutionizing the way we approach semiconductor inspections. The shift from traditional methods to AI-driven techniques is not just a leap in technology; it’s a strategic move to enhance efficiency and accuracy while reducing costs and labor intensity.
Deep learning, particularly through the use of convolutional neural networks (CNNs), has shown promising results in computer vision applications within the industry. These models excel in adapting to complex environments and various chip types, offering a glimpse into a future where real-time defect detection is the norm. This advancement is expected to yield higher efficiency and accuracy, providing a robust support system for the industry’s rapid development.
However, the journey towards fully AI-integrated inspections is not without its challenges. The vital confidentiality of semiconductor data poses a significant hurdle, limiting research and development in this area. Despite this, the potential benefits of AI in semiconductor inspections are too substantial to ignore, and the industry is actively seeking ways to balance confidentiality with the need for advanced research. As we look ahead, the role of AI in semiconductor inspections is poised to unlock unprecedented value, driving innovation and ensuring the production of high-quality chips.
Conclusion
In summary, wafer semiconductor technology is a cornerstone of modern electronics, underpinning the functionality and advancement of devices across various industries. From high-performance computing to electric vehicles, the precision and quality of semiconductor chips are paramount. The shift from traditional manual inspection to advanced methods like deep learning reflects the industry’s need for higher efficiency, accuracy, and adaptability in defect detection. Despite challenges such as data confidentiality, innovations like the DSCU-Net demonstrate the ongoing evolution and potential for more sophisticated semiconductor manufacturing processes. As we continue to push the boundaries of what’s possible with integrated circuits, the role of wafer semiconductor technology will only grow more critical, driving forward the intelligent manufacturing and electronic information technology fields.
Frequently Asked Questions
What challenges does traditional manual visual inspection face in semiconductor defect detection?
Traditional manual visual inspection struggles with low efficiency, low accuracy, high cost, high labor intensity, and inconsistent standards, which limit its application in modern semiconductor production.
How has intelligent manufacturing impacted the semiconductor chip manufacturing process?
Intelligent manufacturing has introduced a complex technology chain that requires precise operation and strict control at every stage, from raw material input to final chip packaging, making integrated circuits increasingly vital in high-end chips.
What role does deep learning play in semiconductor defect detection?
Deep learning algorithms can automatically learn to identify various defects through data training, improving the accuracy and efficiency of detection and adapting to complex environments and different chip types.
Why is there less research on wafer defect detection despite technological advancements?
The vital confidentiality of semiconductor data and other factors have led to limited research in wafer defect detection, despite the potential improvements that could be made with more study.
What are the potential applications of semiconductors in electric vehicles (EVs)?
Semiconductors in EVs are projected to be used extensively in safety, electrification, communication, and networking, with the potential to transform the semiconductor industry and create job opportunities.
How might the proposed novel network structure, DSCU-Net, enhance wafer defect detection?
The proposed DSCU-Net, based on a code and decoder structure, includes an encoder, decoder, and skip connection arranged symmetrically to improve the detection of wafer defects by learning and predicting defect masks more accurately.