Innovation in Automation: The Latest in Semiconductor Manufacturing Machines
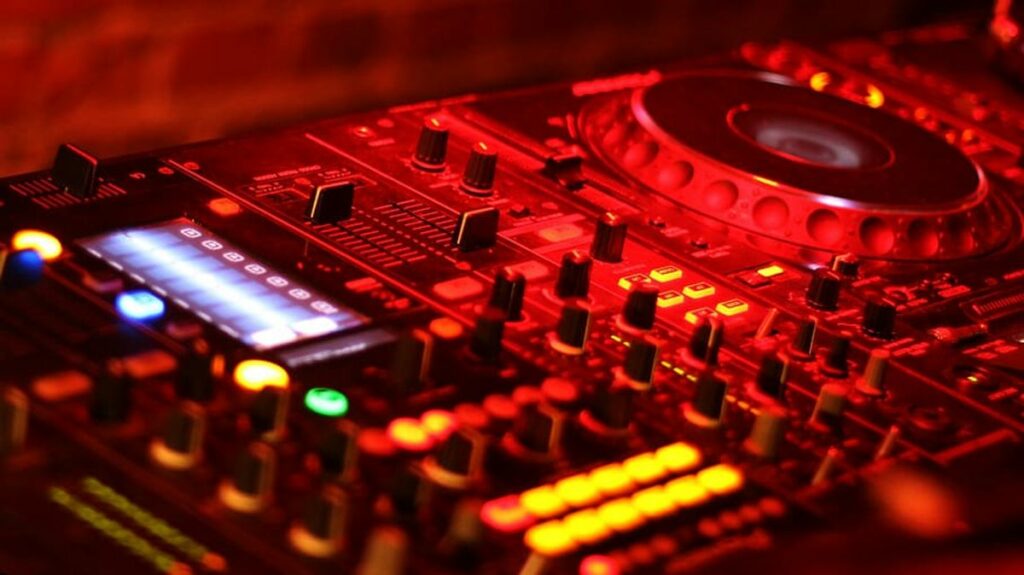
The semiconductor industry is witnessing a transformative era where automation is reshaping every aspect of manufacturing. From wafer handling to yield management, the integration of advanced technologies such as AI and robotics is not only enhancing efficiency and safety but also providing a significant competitive advantage. This article delves into the latest innovations in semiconductor manufacturing machines, exploring how they are revolutionizing the industry, and what future prospects they hold.
Key Takeaways
- Innovative wafer handling automation is crucial for improving accuracy and output, providing a competitive edge in the semiconductor industry.
- The integration of AI and machine learning is revolutionizing semiconductor processes, quality control, and predictive maintenance.
- Smart factories are emerging as a game-changer, optimizing production and impacting industry dynamics through integrated automation.
- Advanced yield management and statistical process control are pivotal in enhancing productivity and ensuring quality in semiconductor manufacturing.
- Automation offers dual benefits of safety and efficiency, reducing human exposure to hazards and streamlining operations with the help of robotics.
Advancements in Wafer Handling Automation
Enhancing Accuracy and Output
In the relentless pursuit of perfection within the semiconductor manufacturing industry, accuracy and output are paramount. The integration of advanced machine learning models, such as ALIGNN, has demonstrated a significant improvement in performance metrics. For instance, when additional training data is incorporated, the Root Mean Square Error (RMSE) values, which are indicative of accuracy, show a marked decrease, implying enhanced precision in wafer handling processes.
The table below illustrates the impact of integrating additional data on the RMSE values for different datasets, highlighting the importance of continuous learning in automation systems:
Dataset | Initial RMSE (eV) | RMSE after Data Integration (eV) |
---|---|---|
Dataset 2 | 2.17 | 0.36 |
Dataset 3 | 2.68 | 0.70 |
Dataset 4 | 2.98 | 0.18 |
This data underscores the critical role of machine learning in driving the future of semiconductor manufacturing. By leveraging such technologies, manufacturers can ensure that their wafer handling systems are not only more accurate but also capable of higher throughput, thus maintaining a competitive edge in an industry where technological advancements are relentless.
The Role of Automation in Competitive Edge
In the high-stakes semiconductor industry, where the pace of innovation is relentless, automation serves as a critical differentiator. Companies that leverage automation can significantly shorten product life cycles, enabling them to introduce new products more swiftly and maintain a competitive stance. This is particularly vital as each advancement in technology nodes brings about a surge in research, design, and production costs.
The integration of automation across the semiconductor value chain has led to a multitude of benefits, including:
- Increased productivity: By reducing cycle times and increasing throughput, automation boosts overall manufacturing efficiency.
- Faster learning ramps: Automation accelerates the onboarding process for new or reskilled technical resources, facilitating quicker adaptation to new technologies.
- Automated metrology and analysis: These tools provide rapid time-to-data, enhancing productivity and enabling more informed decision-making.
Manufacturers that embrace wafer handling automation are poised to secure a substantial competitive advantage. As automation technology continues to evolve, its role in shaping the industry’s future becomes increasingly pronounced, promising even greater gains in efficiency, precision, and safety.
Future Prospects in Wafer Handling Technology
As semiconductor manufacturing continues to evolve, the future of wafer handling automation holds promising advancements. Data analytics are set to revolutionize the capabilities of automated systems, enabling more sophisticated decision-making and efficiency improvements.
Innovations in robotics and sensor technology are anticipated to further enhance the precision and efficiency of wafer handling. These advancements will not only streamline production but also contribute to the overall yield and quality of semiconductor products. The integration of machine learning and AI will facilitate real-time monitoring and predictive maintenance, ensuring optimal production uptime.
The following list highlights key areas of focus for future development in wafer handling technology:
- Advancements in data analytics for improved system capabilities
- Robotics technology enhancements for greater automation precision
- Sensor technology improvements for efficient wafer handling
- Integration of AI for adaptive control and predictive maintenance
Manufacturers that leverage these technological improvements will secure a significant competitive edge, as the industry demands ever-greater levels of accuracy and efficiency.
The Integration of AI and Machine Learning
Smart Algorithms in Semiconductor Processes
The semiconductor industry is witnessing a transformative phase with the integration of smart algorithms, which are pivotal in enhancing the efficiency and precision of manufacturing processes. Smart algorithms are revolutionizing the way semiconductor companies approach design and production, optimizing operations and significantly reducing time to market.
Smart algorithms assist in various stages of semiconductor production, from initial design to final testing. They enable the automation of complex tasks, such as predictive analytics for equipment maintenance, and real-time adjustments to production parameters to ensure optimal performance. The table below highlights the key areas where smart algorithms are applied:
Stage of Production | Application of Smart Algorithms |
---|---|
Design | Accelerated chip design processes |
Fabrication | Real-time process optimization |
Testing | Enhanced accuracy in defect detection |
Maintenance | Predictive analytics for equipment upkeep |
By leveraging AI and machine learning, semiconductor companies are not only improving their operational efficiency but also gaining a competitive edge in a market where innovation and speed are critical. The adoption of these technologies is a clear indicator of a future where smart factories and automated processes become the standard in semiconductor manufacturing.
AI-Driven Quality Control and Metrology
The integration of artificial intelligence (AI) and machine learning (ML) into quality control and metrology has marked a significant shift in semiconductor manufacturing. The value of AI/ML is clear to everyone in the industry, transforming traditional processes into dynamic, predictive systems. Inline inspection, now more than ever, is pivotal for maintaining the high standards required in semiconductor production.
The transition from using measuring laboratories to inline inspection systems is a testament to the industry’s evolution. As we embrace Industry 4.0, the role of Coordinate Measuring Machines (CMMs) is being redefined, ensuring precision and quality at the heart of manufacturing. These advancements are not just about maintaining quality but also about enhancing the capabilities of metrology to meet the demands of modern production scales.
AI-driven systems are capable of analyzing vast amounts of data, leading to more accurate and timely decision-making. This is crucial in an industry where the slightest deviations can lead to significant losses. The table below illustrates the improvements in key performance indicators (KPIs) after implementing AI in metrology processes:
KPI | Before AI | After AI |
---|---|---|
Inspection Time | 30 min | 10 min |
Error Rate | 5% | 0.5% |
Throughput | 100 units/hr | 150 units/hr |
The future trends in precision engineering suggest that the integration of AI will continue to redefine the landscape of quality control and metrology, with robotic assistance playing a crucial role in meeting the ever-increasing demands of the semiconductor industry.
Machine Learning for Predictive Maintenance
The application of machine learning (ML) in predictive maintenance within semiconductor manufacturing is revolutionizing the way facilities approach equipment upkeep and repair. By leveraging comprehensive datasets and advanced algorithms, ML models can predict equipment failures before they occur, reducing downtime and maintenance costs. This predictive capability is particularly crucial in an industry where precision and reliability are paramount.
For instance, a study demonstrated that Graph Neural Networks (GNNs), when trained on extensive defect data, could achieve a Root Mean Square Error (RMSE) as low as 0.32 eV in predicting defect energetics. This level of accuracy in prediction allows for more timely and cost-effective maintenance interventions. The table below summarizes the RMSE achieved for different defect types using ML models:
Defect Type | Data Volume | RMSE (eV) |
---|---|---|
Substitution | ~4553 | 0.36 |
Interstitial | ~12558 | 0.42 |
Combined Model | Sufficient | 0.32 |
The success of ML in predictive maintenance hinges on the quality and comprehensiveness of the training data. It is essential that the data accurately represent the various defect types to ensure the models can generalize their predictive power across different scenarios. As semiconductor manufacturing continues to evolve, the integration of ML for predictive maintenance is set to become a standard, driving efficiency and minimizing disruptions in production.
The Emergence of Smart Factories in Semiconductor Manufacturing
Optimized Production through Integrated Automation
In the quest for optimized production, semiconductor manufacturers are turning to integrated automation as a pivotal solution. Automation is not just a tool but a transformative force that can lead to increased productivity, quality, and consistency across the manufacturing process.
Key benefits of integrated automation include:
- Uniform wafer handling and placement, minimizing errors and enhancing yield rates.
- Cost savings through maximized resource utilization and streamlined procedures.
- Improved safety by reducing manual intervention in hazardous environments.
The challenge lies in balancing productivity with quality, while also preparing for stable production across multiple sites. This is particularly crucial as the industry moves towards less dependence on human interaction and more on digital transformation and AI. By embracing automation, semiconductor factories are setting the stage for a future where supply chains are duplicated and production systems are resilient and adaptable.
The Impact of Smart Factories on Industry Dynamics
The advent of smart factories is revolutionizing the semiconductor industry, altering the dynamics of production and competition. Smart factories are not just a technological leap; they represent a paradigm shift in how semiconductor products are manufactured and delivered. These factories are equipped with advanced automation systems that enable them to self-optimize production processes, leading to significant improvements in efficiency and output.
Smart factories also play a crucial role in the competitive landscape of the semiconductor industry. Manufacturers that harness the power of integrated automation and data analytics are poised to outperform their peers. The table below illustrates the competitive advantages gained by adopting smart factory technologies:
Factor | Advantage |
---|---|
Production Efficiency | Increased throughput |
Quality Control | Enhanced precision |
Supply Chain Management | Streamlined logistics |
Innovation Cycle | Accelerated development |
Looking ahead, the influence of smart factories is expected to grow, with continuous advancements in automation and data analytics further pushing the boundaries of what’s possible in semiconductor manufacturing.
Achieving a Competitive Advantage with Smart Manufacturing
In the semiconductor industry, smart manufacturing stands as a pivotal factor for companies aiming to secure a competitive advantage. By integrating advanced automation and data analytics, manufacturers can streamline their production processes, leading to significant improvements in both efficiency and product quality.
The adoption of smart manufacturing techniques allows for real-time monitoring and control, which in turn enables proactive adjustments and optimization of manufacturing operations. This level of agility and responsiveness is crucial in an industry where demand can fluctuate and precision is paramount.
To illustrate the benefits of smart manufacturing, consider the following points:
- Enhanced production efficiency through automation
- Improved product quality with real-time monitoring
- Increased responsiveness to market demands
- Reduction in operational costs due to optimized processes
Manufacturers that successfully implement these strategies not only improve their operational metrics but also position themselves favorably in a market that values collective success.
Yield Management and Statistical Process Control
Improving Productivity with Advanced Yield Management
Advanced yield management systems are pivotal in the semiconductor manufacturing industry, where the challenge to achieve both productivity and quality is ever-present. By leveraging sophisticated software solutions like yieldWerx, manufacturers can optimize their processes to maximize yield rates and enhance overall operational efficiency.
Key benefits of advanced yield management include:
- Increased productivity through reduced cycle times and higher throughput.
- Faster learning ramps, allowing for quicker integration of new or reskilled technical resources.
- Enhanced capabilities in automated metrology and analysis, leading to faster time-to-data and improved process control.
These systems play a crucial role in stable production across multiple sites, preparing manufacturers for supply chain duplication and reducing reliance on human intervention. As the industry moves towards more integrated and automated solutions, yield management software becomes an indispensable tool for maintaining a competitive edge.
The Importance of Statistical Process Control in Quality Assurance
Statistical Process Control (SPC) is fundamental in maintaining the integrity of semiconductor manufacturing processes. By employing statistical methods, SPC enables manufacturers to monitor and control production quality, ensuring that each semiconductor meets the required standards. This proactive approach to quality assurance is critical in an industry where precision is paramount.
The implementation of SPC involves various techniques, such as control charts, which track the performance of production processes over time. These charts help identify trends and potential issues before they become significant problems, allowing for timely interventions. The table below illustrates a simplified example of how control chart data might be structured:
Time Interval | Measurement | Upper Control Limit (UCL) | Lower Control Limit (LCL) | Status |
---|---|---|---|---|
08:00 | 200.5 | 205.0 | 195.0 | OK |
08:15 | 201.2 | 205.0 | 195.0 | OK |
08:30 | 199.8 | 205.0 | 195.0 | OK |
08:45 | 202.5 | 205.0 | 195.0 | Alert |
In addition to control charts, SPC utilizes other tools such as histograms and Pareto charts to analyze data and drive quality improvements. These tools are part of a broader strategy to leverage data for enhanced semiconductor manufacturing, ultimately leading to higher yields and better product quality.
Leveraging Data for Enhanced Semiconductor Manufacturing
In the realm of semiconductor manufacturing, the strategic use of data is revolutionizing the industry. By harnessing the power of data analytics, companies are able to refine their manufacturing processes, leading to significant improvements in both efficiency and yield. This data-driven approach enables the identification of patterns and anomalies that would otherwise go unnoticed, allowing for proactive adjustments and enhancements.
The integration of data analytics into semiconductor testing is particularly impactful. As highlighted in a recent article titled ‘The Future of Data Analytics and Semiconductor Testing’, the application of AI algorithms and machine learning (ML) is optimizing silicon design, assembly, and testing processes. The analysis of vast amounts of data not only accelerates development cycles but also ensures higher quality outputs.
To illustrate the benefits of leveraging data, consider the following table which outlines key performance indicators (KPIs) before and after the implementation of advanced data analytics:
KPI | Before Data Analytics | After Data Analytics |
---|---|---|
Yield Rate (%) | 85 | 92 |
Defect Density (defects/cm^2) | 0.02 | 0.015 |
Cycle Time (days) | 30 | 25 |
This table clearly shows the tangible improvements in yield rate, defect density, and cycle time, underscoring the critical role of data in enhancing semiconductor manufacturing processes.
Safety and Efficiency: The Dual Benefits of Automation
Reducing Human Exposure to Hazardous Conditions
The semiconductor manufacturing industry is witnessing a significant shift towards automation, particularly in areas that pose potential risks to human workers. Safeguarding the human factor is a critical aspect of this transition. Semiconductor fabrication often involves tasks that are repetitive, ergonomically challenging, or potentially hazardous. By introducing collaborative robots (cobots), companies are not only enhancing efficiency but also revolutionizing cleanroom environments.
Automation systems are now designed to ensure uniform wafer handling and placement, which is crucial for maintaining high yield rates. This precision reduces the likelihood of errors that can occur with manual processes. Moreover, automation plays a pivotal role in cost savings, as it maximizes resource utilization and streamlines procedures, leading to increased profitability.
The integration of advanced safety measures through automation has led to a reduction in the need for manual intervention in dangerous situations. This not only enhances security for the workforce but also contributes to the stabilization of production quality. The table below summarizes the benefits of automation in reducing human exposure to hazardous conditions in semiconductor manufacturing:
Benefit | Description |
---|---|
Enhanced Safety | Reduces the need for human presence in high-risk areas. |
Quality Stabilization | Maintains consistent handling and placement of wafers. |
Cost Efficiency | Lowers manufacturing expenses by optimizing resource usage. |
Streamlining Operations for Greater Efficiency
In the fast-paced world of semiconductor manufacturing, streamlining operations is a critical factor in driving greater efficiency. Automation plays a pivotal role in this transformation, enabling manufacturers to optimize every aspect of their production process. By reducing cycle times and increasing throughput, automation not only enhances productivity but also contributes to an accelerated market launch.
Key benefits of automation in streamlining operations include:
- Increased productivity: By minimizing manual intervention, automation allows for continuous, efficient production runs.
- Faster learning ramps: New technologies and processes can be integrated and mastered more quickly, thanks to automated systems.
- Automated metrology and analysis: These systems provide rapid, accurate data, facilitating timely decision-making and process adjustments.
Furthermore, automation is instrumental in future-proofing semiconductor manufacturing. It ensures that operations can adapt to evolving technologies and market demands, maintaining a competitive edge. The integration of smart algorithms and machine learning into semiconductor manufacturing processes significantly enhances efficiency, paving the way for more sophisticated advancements in the industry.
The Role of Robotics in Ensuring Workplace Safety
In the semiconductor manufacturing industry, robots are pivotal in minimizing human exposure to hazardous conditions. Some stages of semiconductor fabrication involve working with materials that may pose risks to human health. By delegating these tasks to robots, companies can ensure a safer work environment while maintaining high efficiency.
Robots not only enhance safety but also bring about significant improvements in precision and accuracy. This is particularly crucial in an industry where the slightest deviation can lead to product defects. The table below summarizes the dual benefits of robotics in semiconductor manufacturing:
Benefit | Description |
---|---|
Safety | Reduces human exposure to hazardous materials and conditions. |
Efficiency | Accelerates production and time to market, while improving precision in component placement. |
Looking ahead, the integration of robotics in semiconductor manufacturing is expected to evolve, with smarter, more autonomous systems taking on increasingly complex tasks. This progression will likely continue to reshape the landscape of workplace safety and operational efficiency in the industry.
Conclusion
The landscape of semiconductor manufacturing is undergoing a transformative shift, with automation playing a pivotal role in shaping the future of the industry. From the integration of AI and machine learning to the rise of smart factories, the advancements in wafer handling automation are not only enhancing productivity and precision but also providing a competitive advantage to those who adopt these technologies. As we have explored, the benefits of automation extend across the entire value chain, offering increased throughput, faster learning ramps for technical resources, and improved yield management. The continued innovation in semiconductor manufacturing machines promises to drive the industry forward, ensuring that manufacturers can meet the ever-increasing demands for efficiency and innovation in an intensely competitive market.
Frequently Asked Questions
How is automation transforming the semiconductor industry?
Automation is transforming the semiconductor industry by enhancing productivity, reducing cycle times, increasing throughput, and improving quality. It enables faster learning ramps for new technologies, provides automated metrology and analysis for quicker time-to-data, and supports rapid innovation to maintain a competitive edge in a fast-paced market.
What are the benefits of wafer handling automation?
Wafer handling automation offers numerous benefits, including increased accuracy and output in semiconductor manufacturing, reduction of human error, improved safety by minimizing human exposure to hazardous conditions, and the potential to gain a competitive advantage through optimized production processes.
How does AI and machine learning integration impact semiconductor manufacturing?
The integration of AI and machine learning in semiconductor manufacturing leads to smarter algorithms for process optimization, AI-driven quality control and metrology for higher accuracy, and predictive maintenance to prevent downtime. This results in enhanced efficiency, reduced waste, and improved overall product quality.
What is the role of smart factories in semiconductor manufacturing?
Smart factories play a crucial role in semiconductor manufacturing by integrating advanced automation technologies to optimize production processes, ensure maximum efficiency, and provide a significant competitive advantage through innovative manufacturing solutions and real-time data analytics.
How does yield management and statistical process control improve semiconductor manufacturing?
Yield management and statistical process control improve semiconductor manufacturing by leveraging data to enhance productivity, ensure quality assurance through rigorous process monitoring, and enable manufacturers to make informed decisions to optimize manufacturing processes and reduce defects.
What are the dual benefits of automation in terms of safety and efficiency?
Automation offers the dual benefits of improving workplace safety by reducing human exposure to dangerous conditions and increasing operational efficiency by streamlining processes, reducing manual intervention, and utilizing robotics to ensure consistent and precise execution of tasks.