Innovations in Wafer Testing Equipment for Enhanced Chip Quality
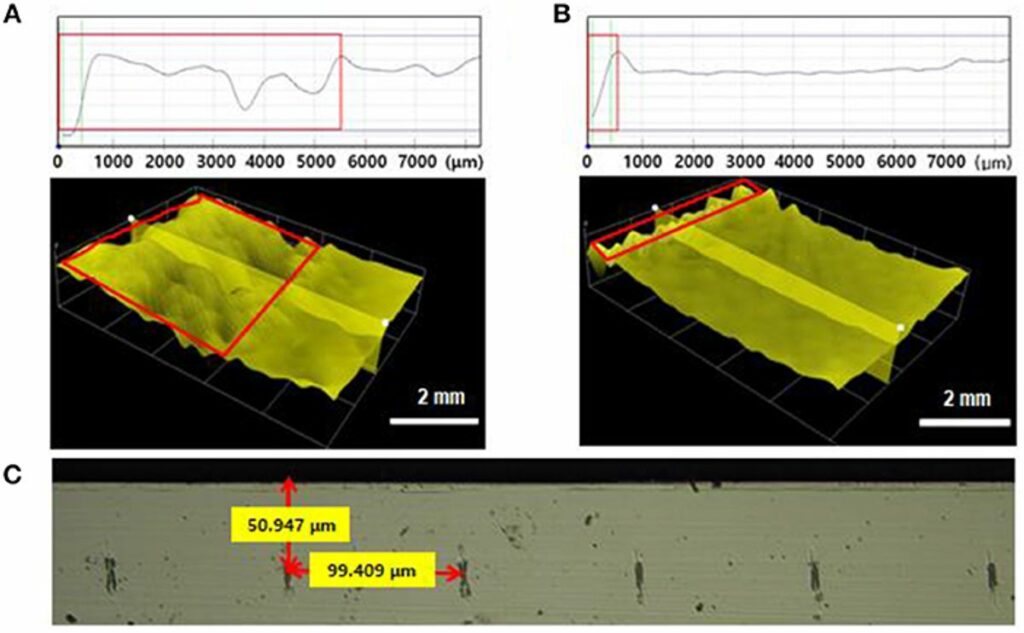
The semiconductor industry is on the cusp of a transformative era with the advent of cutting-edge wafer testing equipment. These innovations are not only enhancing chip quality but also redefining the standards of efficiency and reliability in chip manufacturing. From in-die monitoring to advanced metrology, and from tackling wafer-level packaging challenges to leveraging data-driven strategies, the sector is witnessing a paradigm shift. This article explores the latest technologies and approaches that are setting new benchmarks in the production of high-quality semiconductor devices.
Key Takeaways
- The integration of in-die monitoring IP and AI/ML models is revolutionizing real-time test decisions, ensuring each chip’s unique ‘fingerprint’ enhances quality control.
- Customization and adaptability in semiconductor test and metrology are key to addressing customer-specific needs and novel issues, supported by robust data management systems.
- Innovative inspection techniques for 3D structures and wire bonds, as well as thermal management strategies for 2.5D integration, are critical in overcoming wafer-level packaging challenges.
- Data provenance and predictive analytics are instrumental in improving final test yields, with knowledge transfer across the supply chain driving continuous improvement.
- Standardization efforts, particularly in chiplet IP, along with industry collaboration and talent development, are essential for sustaining innovation and growth in the chip industry.
Emerging Technologies in In-Die Monitoring
In-Die Monitoring IP for Chip Fingerprinting
The integration of In-Die Monitoring IP is a pivotal step towards ensuring chip uniqueness and traceability throughout its lifecycle. Unique identifiers, akin to fingerprints for semiconductor devices, are embedded early in the design phase and persist through production, enabling a new level of quality assurance and supply chain security.
To achieve this, a multi-faceted approach is essential, encompassing:
- In-die monitoring IP that provides accurate chip fingerprints;
- Centralized analytics platforms for comprehensive data integration;
- Advanced analytics for training AI/ML models, which are then integrated back into the test program for real-time decision-making during test phases.
This technology not only plugs gaps in the integrated circuit (IC) supply chain but also enhances the ability to track and verify chips at every stage, from design to end-use.
Centralized Analytics Platforms for Data Integration
The semiconductor industry is witnessing a transformative shift with the integration of centralized analytics platforms that can absorb and process vast amounts of data. These platforms are crucial for enabling data preparation and engineering capabilities, which in turn, support the training of AI/ML models. By streamlining these models back into the test program, each chip in production can be evaluated in real-time, leading to more informed decisions during the test phase.
One of the key benefits of these analytics platforms is the democratization of data within the fabrication plants. Complex datasets are distilled into actionable insights, making them accessible to a broader range of stakeholders. This shift ensures that decision-making is a collective effort, rather than being limited to a select group of data scientists or engineers.
However, the integration of AI/ML into semiconductor fabs is not without its challenges. Fabs must address the quality and relevance of data, the computational demands of processing large datasets, and the cultural shift towards embracing advanced technologies. Moreover, the industry’s reluctance to share data due to competitive concerns creates a barrier to maximizing the potential of AI/ML. To overcome this, the development of industry standards for AI/ML models is essential. Such standards would provide a framework for data generation, sharing, and utilization, fostering a collective intelligence pool without risking proprietary information.
AI/ML Model Training for Real-Time Test Decisions
The integration of AI/ML in wafer testing equipment is revolutionizing the way real-time decisions are made during the chip quality assurance process. AI/ML models must navigate decision-making thresholds with precision, ensuring that only genuine concerns are flagged without being overwhelmed by data variances. This precision is crucial to maintain system stability and avoid unnecessary disruptions.
In practice, setting the right thresholds for AI/ML models is a complex task. It involves a careful balance of various factors and a deep understanding of the objectives to be optimized. Manufacturers are approaching the integration of these models with caution, often running extensive tests within known parameters before full deployment. The consensus is that it’s better to deal with false failures than to miss defects, as the latter can have more severe consequences.
The challenge for AI/ML research is to develop models capable of identifying unexpected issues, even those not previously encountered. This requires models that are not only predictive but also possess the ability to recall, compare, and reason. The table below summarizes the key considerations for AI/ML model training in wafer testing:
Consideration | Description |
---|---|
Precision | Models must accurately distinguish between pass and fail outcomes. |
Stability | Models should maintain system stability without causing disruptions. |
Threshold Setting | Careful balance of factors is needed to set appropriate decision-making thresholds. |
Testing | Models are thoroughly tested within known parameters before full integration. |
False Positives vs. Missed Defects | A preference for false failures over missed defects to prevent severe consequences. |
Adaptability | Models must quickly adjust to changes and handle unknowns efficiently. |
Advancements in Semiconductor Test and Metrology
Adapting to Customer-Specific Needs
In the realm of semiconductor test and metrology, the ability to adapt to customer-specific needs is paramount. Flexibility in testing approaches is essential to cater to the unique requirements of each chip design. This often involves customizing test equipment and methodologies to ensure that all performance parameters are met. For instance, the integration of machine learning models into the testing process can vary significantly depending on the data and its distribution, necessitating a tailored approach for each scenario.
To achieve this, a robust support system is crucial. It must be capable of managing the vast amounts of data generated during testing and ensuring its readiness for analysis. The following points highlight the key aspects of such a system:
- Seamless integration of testing data into the manufacturing flow
- Efficient data collection and management to facilitate machine learning model training
- Adaptability to prevent overfitting of models to specific design scenarios
By addressing these points, manufacturers can unlock improvements in yields, process optimization, and defect reduction, ultimately enhancing the overall quality of semiconductor devices.
Identifying and Addressing Novel Issues
As the semiconductor industry pushes the boundaries of technology, novel issues in wafer testing are inevitable. These challenges often arise from the introduction of new materials, complex architectures, and the miniaturization of components. To maintain the balance between efficiency and sensitivity, especially in the defect inspection of non-patterned wafer production lines, a multi-faceted approach is required.
One such approach involves the enhancement of existing inspection methodologies. For instance, the integration of advanced imaging techniques with machine learning algorithms can lead to the early detection of defects that were previously undetectable. The table below summarizes the improvements in defect detection rates with the adoption of new technologies:
Technology | Previous Detection Rate | Improved Detection Rate |
---|---|---|
Advanced Imaging | 70% | 85% |
Machine Learning | 65% | 90% |
Furthermore, the industry is moving towards a more collaborative model, where sharing insights and data across different stages of the semiconductor lifecycle can preemptively address potential issues. This includes establishing standards for data exchange and fostering partnerships between equipment manufacturers, chip designers, and foundries.
Support Systems for Comprehensive Data Management
In the pursuit of enhanced chip quality, semiconductor fabs are turning to sophisticated support systems for comprehensive data management. The integration of AI/ML technologies is pivotal in managing the deluge of data generated across various manufacturing stages. These systems are not only tasked with data collection but also with ensuring its traceability and quality throughout the chip’s lifecycle.
The challenges in implementing these systems are multifaceted. They include overcoming siloed data across departments, compatibility issues between different equipment manufacturers, and the precise adjustment of sensors and machines for accurate data capture. Here is a summary of the key integration challenges:
- Quality and relevance of data for AI models
- Computational demands for processing large datasets
- Cultural shift towards embracing advanced technologies
By addressing these challenges, fabs can leverage AI/ML to democratize data access, enabling stakeholders with varying levels of technical expertise to engage in informed decision-making. This collective approach is essential for rapid interpretation and decisive action, which are critical for maintaining a competitive edge in the semiconductor industry.
Challenges and Solutions in Wafer-Level Packaging
3D Structures and Wire Bond Inspection
The inspection of 3D structures and wire bonds is critical for ensuring the reliability and performance of semiconductor devices. Advanced imaging techniques are employed to scrutinize surface textures and detect potential irregularities that may arise during the molding process. These techniques are essential for identifying defects that could compromise the integrity of wire bonds and the overall device functionality.
Bonding surface analysis has become a focal point in quality control, with various substrates undergoing detailed examination:
- FR-4 Substrate: Characterized by consistent pitting, with roughness measurements around 1 \
Thermal Integrity in 2.5D Integration
Maintaining thermal integrity during 2.5D integration is crucial for ensuring the reliability and performance of semiconductor devices. The challenge lies in effectively dissipating heat from densely packed components without compromising the structural integrity of the wafer. This is particularly important as devices become more complex and power densities increase.
Several strategies have been developed to address thermal management in 2.5D integration:
- Utilization of high thermal conductivity materials such as copper and silicon carbide.
- Implementation of microfluidic cooling channels within the interposer layer.
- Design of efficient thermal interface materials (TIMs) to enhance heat transfer.
The table below summarizes the thermal conductivity of common materials used in 2.5D integration:
Material | Thermal Conductivity (W/mK) |
---|---|
Copper | 385 |
Silicon Carbide | 490 |
Diamond | 2000 |
Standard TIMs | 5 – 10 |
Advancements in material science and cooling techniques are essential for overcoming the thermal challenges associated with next-generation semiconductor devices. Collaboration between industry experts and researchers is key to developing innovative solutions that can be integrated into the manufacturing process.
Hybrid Bonding Techniques for Next-Gen Devices
The semiconductor industry is rapidly advancing with the adoption of hybrid bonding techniques for next-generation devices. This method, known for its ability to stack multiple heterogeneous chips, is becoming increasingly important due to the high 3D interconnect density it offers. Hybrid bonding, particularly wafer-to-wafer hybrid bonding, is recognized for its potential in 3D integration technology.
One of the key advantages of hybrid bonding is the precision it brings to the table. By allowing for the direct bonding of wafers without the need for an intermediary layer, it significantly reduces the overall thickness of the stack and improves the performance of the final chip. The table below outlines some of the critical aspects of hybrid bonding compared to traditional methods:
Bonding Technique | Interconnect Density | Layer Thickness | Performance Impact |
---|---|---|---|
Traditional | Low | Thicker | Lower |
Hybrid | High | Thinner | Higher |
As the industry moves towards more complex and miniaturized devices, the role of hybrid bonding will only grow in significance. It is essential for manufacturers to continue refining this technique to meet the evolving demands of the market.
Data-Driven Approaches to Enhance Chip Quality
Traceability and Data Provenance in the Semiconductor Lifecycle
In the semiconductor industry, traceability and data provenance are foundational to the integrity of AI-driven analytics. These elements enable manufacturers to not only respond to production anomalies but also to predict and prevent them, establishing a feedback loop that enhances machine learning models through continuous improvement.
The importance of traceability extends to the protection of intellectual property, which is crucial for maintaining market competitiveness. However, the industry’s fragmented data landscape often results in isolated data models, hindering the potential for a unified, intelligent supply chain.
To address these challenges, the industry is moving towards solutions that foster trust and compliance while safeguarding proprietary information. The table below outlines the key aspects of traceability and data provenance that are critical for the future of semiconductor manufacturing:
Aspect | Description |
---|---|
Data Integration | Ensuring data from the entire supply chain is integrated for improved yield prediction. |
Feedback Loop | Facilitating traceability of outcomes to specific data inputs for model learning. |
IP Protection | Balancing the need for open data sharing with the protection of intellectual property. |
Operational Efficiency | Linking interconnected data for insightful analytics in chiplet and integrated device production. |
As the industry advances, particularly in the realm of chiplets, the interconnectedness of data will become increasingly vital for insightful analytics and operational efficiency. The challenge lies in finding practical solutions that balance IP protection with the imperative for collective process improvement.
Predictive Analytics for Final Test Yield Improvement
The integration of predictive analytics into wafer testing represents a significant leap forward in ensuring chip quality. Predictive models, informed by diverse data sets, can forecast potential failures, allowing for preemptive measures to be taken. This not only prevents costly production delays but also extends the lifespan of testing equipment, optimizing both investment and resource allocation.
Predictive analytics leverages machine learning (ML) to analyze vast amounts of data, including upstream information, to predict downstream failures. For instance, a high failure rate in a specific area of the wafer can indicate an increased likelihood of defects in the surrounding good dies. By employing ML, fabs can adopt methodologies that yield more accurate failure predictions.
To effectively implement predictive analytics, a fab must not only collect extensive data but also ensure its traceability and quality throughout the semiconductor lifecycle. Integrated data from the entire supply chain leads to more precise final test yield predictions. The table below illustrates the impact of predictive analytics on yield improvement:
Metric | Before Predictive Analytics | After Predictive Analytics |
---|---|---|
Yield Improvement | 2% | 5% |
Production Downtime | 10% | 5% |
Equipment Lifespan | 3 years | 4 years |
The success of AI/ML in predictive analytics hinges on the ability to learn from both the back end and front end of the production process. However, only a select number of companies have managed to integrate this knowledge effectively.
Knowledge Transfer Across the Semiconductor Supply Chain
The semiconductor industry is recognizing the importance of knowledge transfer as a vital component for maintaining a competitive edge. A Large Education Gap and Poor Knowledge Transfer have been identified as key issues in the industry, particularly in the context of the skills shortage. To address this, companies are increasingly focusing on the flow of information and expertise throughout the supply chain.
One of the primary methods to facilitate knowledge transfer is through the creation of collaborative environments. These spaces allow for the sharing of insights and best practices among industry players, from foundries to fabless companies. However, historical defensive postures can impede progress, making it essential to foster a culture of openness and cooperation.
To illustrate the current state of knowledge sharing in the semiconductor industry, consider the following table of industry experts and their contributions to the dialogue:
Expert | Focus Area |
---|---|
David Leary | Improving Chip Reliability |
Ann Mutschler | Supply Chain Security |
Cliff Greenberg | Industry Standards |
Kevin Parmenter | Supply Chain Threats |
Esther Soria | Tech Collaboration in Automotive |
Kumar Venkatramani | Semiconductor Predictions |
By leveraging the expertise of such individuals and integrating lessons learned across the supply chain, companies can enhance their AI/ML capabilities, leading to better predictive outcomes for final test yields.
Standardization and Collaboration in the Chip Industry
The Role of Chiplet IP Standards
The integration of chiplets into a single device architecture is revolutionizing the semiconductor industry, but it also introduces complex challenges in intellectual property (IP) management. Standardization of Chiplet IP is crucial for ensuring interoperability and fostering innovation while protecting the proprietary technologies of different stakeholders.
To address these challenges, the industry is moving towards the development of common standards that define interfaces, protocols, and compliance tests. This collaborative effort aims to streamline the integration process and reduce time-to-market for new devices. The following points highlight the key aspects of chiplet IP standardization:
- Development of open interface standards for seamless chiplet integration
- Compliance mechanisms to ensure interoperability across different vendors
- Protection of proprietary IP while enabling data sharing for collective improvement
- Establishment of trust among industry players through transparent practices
As noted by experts like Dr. Dev Gupta and Erik Jan Marinissen, the future of chiplets depends on finding a balance between open collaboration and IP security. The industry’s commitment to standardization is a testament to its dedication to overcoming these challenges and advancing the capabilities of integrated devices.
Cross-Industry Apprenticeships and Talent Development
The semiconductor industry is facing a significant talent gap, which is widening as the technology becomes more complex and the demand for skilled workers increases. Companies will need to cast a wider net to attract and retain the necessary talent to drive innovation and maintain competitive advantage. Improving the employee value proposition is crucial, as is maximizing the productivity of the existing workforce.
To address these challenges, cross-industry apprenticeships and talent development programs are emerging as a strategic solution. These initiatives aim to foster a continuous learning environment and facilitate knowledge transfer across different sectors of the industry. For example, early STEM education initiatives and partnerships with academic institutions are critical steps in cultivating a future-ready chip workforce.
The table below outlines some key figures and entities contributing to the dialogue on talent development within the industry:
Contributor | Focus Area |
---|---|
Mark Camenzind | IC Industry Work Culture |
Liz Allan | Early STEM Education |
Art Scott | Engineering Education Reform |
Rob Pearson – RIT | STEM Workforce Growth |
By investing in these programs, companies not only bridge the talent divide but also ensure a robust pipeline of skilled professionals equipped to handle the challenges of next-generation chip development.
Collaborative Efforts for Mixed-Foundry Chiplets
The commercial chiplet ecosystem is on the horizon, yet it may take a decade to fully realize the vision of a seamless, LEGO-like assembly of chiplets. Despite the aspirational nature of this concept, business impediments currently stand in the way. The industry is actively working to overcome these challenges through collaboration and standardization.
Collaborative efforts are essential for the development of mixed-foundry chiplets. These efforts involve multiple stakeholders, including IP providers, foundries, and end-users, who must work together to ensure compatibility and performance across different manufacturing platforms. Key to this collaboration is the establishment of trust and the implementation of compliance mechanisms that allow for the sharing of data without compromising proprietary secrets.
To facilitate this, the industry is exploring various strategies:
- Developing common standards for chiplet interfaces
- Creating frameworks for IP protection that enable data sharing
- Encouraging cross-industry partnerships to leverage diverse expertise
As these strategies are put into practice, the industry moves closer to a future where chiplets can be mixed and matched with greater ease, driving innovation and efficiency in chip design and manufacturing.
Conclusion
The advancements in wafer testing equipment are pivotal for the semiconductor industry’s pursuit of impeccable chip quality. Innovations such as in-die monitoring IP, centralized analytics platforms, and AI/ML-driven analytics capabilities are revolutionizing the way chips are tested and monitored. These technologies not only enhance the detection of defects but also contribute to the optimization of manufacturing processes. The ability to adapt to customer-specific needs while identifying new issues is crucial, and this is made possible by a robust support system that manages data effectively from inception to completion. As industry experts like Advantest’s Leventhal and Bachiraju have highlighted, the integration of comprehensive data and traceability systems is essential for predictive analytics and AI/ML success. The future of semiconductor testing is bright, with these innovations leading to more reliable, efficient, and high-quality chip production.
Frequently Asked Questions
What is in-die monitoring IP and how does it contribute to chip fingerprinting?
In-die monitoring IP refers to integrated intellectual property that operates within a chip to monitor its performance and characteristics. It can provide a unique ‘fingerprint’ for each chip, identifying it and its behavior, which is crucial for optimization and quality control in semiconductor manufacturing.
How do centralized analytics platforms improve semiconductor testing?
Centralized analytics platforms collect and integrate data from various sources, enabling better data preparation and engineering. This facilitates the training of AI/ML models, which can then be used to make real-time decisions during chip testing, thereby enhancing the test process.
What challenges do 3D structures pose for wire bond inspection?
3D structures can complicate wire bond inspection due to their complexity and the difficulty in accessing and visualizing the bonds. Advanced inspection techniques are required to ensure the integrity of these connections in such densely packed environments.
How does thermal integrity affect 2.5D integration, and what are the solutions?
Thermal integrity is crucial in 2.5D integration as it ensures that heat is managed effectively to prevent damage and maintain performance. Solutions include advanced materials and design techniques that improve heat dissipation and maintain the structural integrity of the chip.
What role do predictive analytics play in semiconductor manufacturing?
Predictive analytics use data from the manufacturing process to forecast outcomes and identify potential issues before they occur. This helps in improving the final test yield and overall chip quality by enabling proactive adjustments in the manufacturing process.
Why is standardization important in the chip industry, particularly for chiplets?
Standardization in the chip industry ensures compatibility and interoperability between different components, such as chiplets. It facilitates collaboration and reduces complexity in the design and manufacturing process, leading to more efficient and scalable production of semiconductor devices.