Silicon Synapses: The Role of Semiconductor Integrated Circuits in Modern Technology
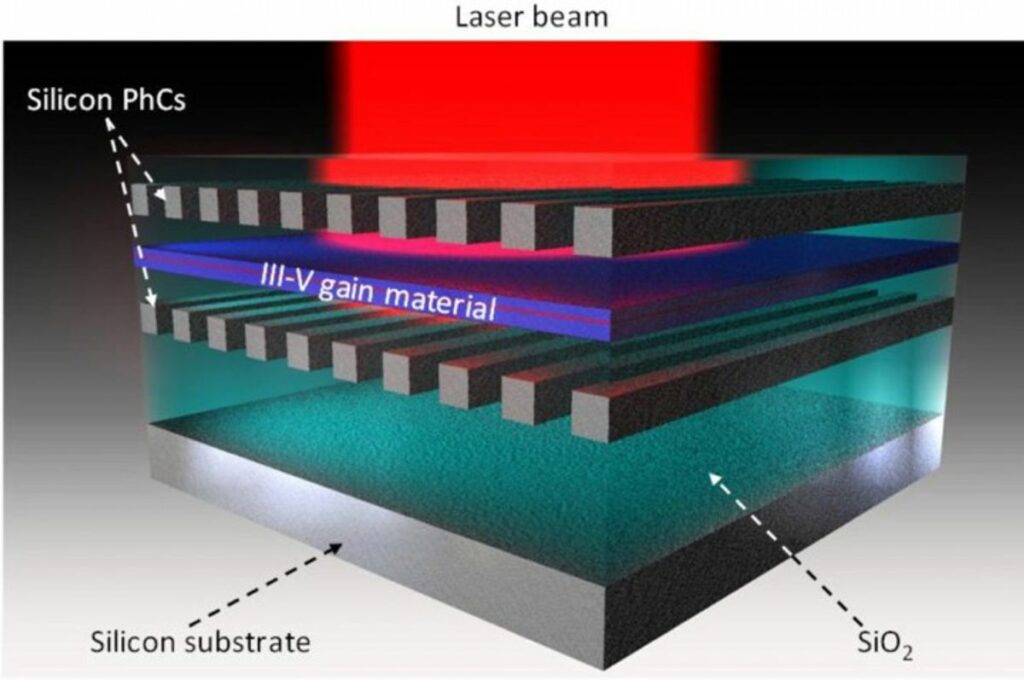
In the article ‘Silicon Synapses: The Role of Semiconductor Integrated Circuits in Modern Technology,’ we delve into the transformative impact of semiconductor technology on the evolution of computing, particularly focusing on the advancements in neuromorphic computing that mimic the human brain’s neural structures. As we approach the physical limits of silicon-based integrated circuits, new materials and quantum effects come into play, reshaping the landscape of modern technology. This exploration covers the journey from Moore’s Law to the cutting-edge of cognitive computing and the future directions of semiconductor integrated circuits.
Key Takeaways
- The quantum effects in silicon-based FETs signal the approaching physical limits of current semiconductor technology, necessitating the exploration of carbon-based semiconductors and post-Moore innovations.
- Neuromorphic computing, which bridges biology and technology through the synapse/neuron model, is seeing increased efficiency with the development of memristive devices and electronic/ionic hybrid materials.
- Advancements in integrated circuit design, such as innovations in CMOS technology and the scaling down of transistors, continue to drive the capabilities of modern computing devices.
- Silicon synapses have a significant impact on cognitive computing, with notable circuits like TrueNorth and Loihi highlighting the potential to replicate human brain capabilities in a cost-effective and efficient manner.
- The future of semiconductor integrated circuits lies in exploring new materials beyond traditional CMOS, the implications of in-sensor processing, and the scalability of neuromorphic hardware.
The Evolution of Semiconductor Technology
From Moore’s Law to Post-Moore Innovations
Since its inception in 1965, Moore’s Law has been a guiding metric for the semiconductor industry, predicting the doubling of transistors on integrated circuits every two years. This trend has led to exponential growth in computational power and miniaturization of electronic devices. However, as we approach the physical limits of silicon-based technology, the industry faces a critical transition period.
The relentless pursuit of Moore’s Law has fueled innovations beyond traditional scaling, giving rise to what some refer to as the ‘Post-Moore’ era. This new phase is characterized by diverse advancements that aim to maintain the pace of progress in computing power. Among these are novel materials, such as high-mobility organics and carbon nanotubes, and alternative computing paradigms like integrated photonics.
- Novel Materials: High-performance organic field-effect transistors, carbon nanotube-based devices.
- Alternative Paradigms: Integrated photonics for high-performance computing.
- AI Demand: Surge in computational power requirements driven by AI and deep learning.
The question of whether Moore’s Law is ‘dead’ has been a topic of debate, with some experts suggesting that we have already entered a slowdown in semiconductor progress. Yet, the industry continues to innovate, finding new ways to enhance performance and functionality of electronic devices.
The Rise of Carbon-Based Semiconductors
As the era of traditional silicon-based semiconductors encounters its physical and economic limits, the exploration of alternative materials has become a pivotal focus in the semiconductor industry. Carbon-based semiconductors have emerged as a promising avenue, offering a new realm of possibilities for electronic devices. Researchers at the Georgia Institute of Technology have made a significant breakthrough by creating the world’s first functional semiconductor made from graphene, a single layer of carbon atoms arranged in a hexagonal lattice.
The advantages of carbon-based semiconductors, such as carbon nanotubes (CNTs) and graphene, are numerous. They exhibit superior electrical transport properties compared to silicon, which could lead to faster and more energy-efficient electronic components. The table below summarizes the key properties that set carbon-based semiconductors apart from their silicon counterparts:
Property | Silicon | Carbon-based Materials |
---|---|---|
Electrical Conductivity | Moderate | High |
Current Density | Lower | Higher |
Heat Dissipation | Less Efficient | More Efficient |
Flexibility | Rigid | Flexible |
Despite these promising attributes, the integration of carbon-based materials into existing manufacturing processes poses significant challenges. The industry must overcome hurdles related to scalability, uniformity, and integration with current silicon-based technology. However, the potential rewards of surmounting these obstacles are substantial, paving the way for the next generation of integrated circuits that could revolutionize computing as we know it.
Quantum Effects in Silicon-Based FETs
The relentless miniaturization of field-effect transistors (FETs) has ushered in quantum mechanical phenomena that cannot be ignored. Quantum interference could lead to smaller, faster, and more energy-efficient devices. However, as transistors shrink, they face increasing inefficiencies and susceptibility to errors. Electrons can leak through the device, even when it should be off, due to quantum tunneling.
Silicon photonics is a promising area where quantum effects are being harnessed. By manipulating the effective refractive index (neff), devices can control light with precision. This is achieved by doping silicon waveguides and applying an external electric field, which tunes the carrier concentration and, consequently, the neff. Such advancements are pivotal for developing silicon photonic-electronic neural networks and other optoelectronic systems.
The integration of quantum mechanics into silicon-based FETs is not without challenges. Scaling down transistors to the quantum realm introduces new complexities in design and fabrication. Here is a brief overview of recent efforts:
- A novel MOSFET with lateral-vertical charge coupling for extremely low gate-drain capacitance (Cgd).
- Harnessing plasma absorption in silicon MOS ring modulators for enhanced performance.
- Development of low-power MOS-capacitor based silicon photonic modulators.
Neuromorphic Computing: Bridging Biology and Technology
The Synapse/Neuron Model in CMOS Circuits
The quest to mimic the human brain’s synaptic and neuronal functions has led to the integration of the synapse/neuron model into CMOS circuits. This endeavor requires a significant number of CMOS transistors, highlighting the inherent inefficiency of CMOS technology when not specifically designed for neuromorphic purposes. Despite this, neuromorphic integrated circuits such as TrueNorth and Loihi have showcased the potential of CMOS in replicating cognitive functions with a degree of energy efficiency.
Neuromorphic devices have evolved to include two-terminal memristive devices and multi-terminal transistor devices, each with its own set of advantages and challenges. Memristors, in particular, offer a promising route for ultra-high integration due to their two-terminal structure, which is conducive to synaptic weight updating and neuronal integrate-fire functions. However, the absence of a select terminal in two-terminal memristors can lead to selectivity issues and unintended sneak path currents.
The following table compares the traditional CMOS approach with device-based neuromorphic systems, emphasizing the potential for compact and energy-efficient computing:
Feature | CMOS IF Neuron Circuit | Device-Based Neuromorphic System |
---|---|---|
Integration Level | Low (tens of transistors) | High (two-terminal structure) |
Energy Efficiency | Moderate | High |
Design Complexity | High | Variable (depends on device) |
Potential for Learning | Limited | Enhanced (STDP circuits) |
These results emphasize the potential of the proposed neuron and STDP learning circuits for compact and energy-efficient neuromorphic computing systems.
Emerging Neuromorphic Devices and Their Efficiencies
The quest for brain-like perception and energy efficiency in electronics has led to the development of neuromorphic devices. These devices are designed to mimic the biological nervous systems and are pivotal in the next era of high-efficiency hardware. Neuromorphic integrated circuits, particularly those using CMOS technology, have been instrumental in replicating the cognitive functions of the human brain.
However, the inherent inefficiencies of CMOS technology for simulating synapses and neurons have spurred the creation of devices specifically tailored for this purpose. The construction of a functional synapse/neuron model in CMOS circuits often requires multiple transistors, leading to increased complexity and energy consumption. To address these challenges, researchers are exploring various innovative approaches:
- Monolithic fabrication processes that promise to streamline the production of neuromorphic devices.
- Integration with advanced CMOS nodes to enhance computational capabilities.
- 3-D electro-optical integration techniques aimed at improving system performance.
Notable neuromorphic devices such as TrueNorth and Loihi have set benchmarks in the field. Yet, the journey towards achieving the efficiency of the human brain’s dense synaptic interconnections continues. The table below summarizes the key attributes of these emerging devices:
Device | Key Feature | Energy Efficiency | Development Stage |
---|---|---|---|
TrueNorth | Cognitive computing | High | Mature |
Loihi | Learning algorithms | Moderate | Experimental |
The evolution of neuromorphic devices is a testament to the relentless pursuit of creating hardware that not only computes but also learns and adapts like the human brain.
Ionic Dynamics and Electronic Hybrid Materials
The intersection of ionic dynamics and electronic materials has given rise to a novel class of devices known as ionic/electronic hybrid materials. These materials are at the forefront of bio-inspired computing, leveraging the unique properties of ions to emulate biological processes. For instance, oxide ionic transistors harness ionic motion to modulate electronic signals, creating a bridge between the digital and biological realms.
Recent studies have highlighted the potential of these materials in neuromorphic applications. The table below summarizes key research findings in this domain:
Reference | Material | Application | Key Findings |
---|---|---|---|
Shi et al., 2023 | Mixed proton and electron conductor | Non-associative learning behavior | Demonstrated in a hybrid pseudo-diode |
Long et al., 2020 | Bio-polymer electrolyte gated oxide | Heterosynaptic mechanisms | Global modulatory effects observed |
Li et al., 2020 | Li-Ion doped In2O3/AlLiO | Memory and learning enhancement | Improved electrical-double-layer modulation |
These advancements suggest a promising direction for the development of neuromorphic systems that can mimic the synaptic functions of the human brain. The integration of ionic dynamics with electronic materials is not only enhancing the capabilities of synaptic transistors but also paving the way for more complex cognitive functions in artificial systems.
Advancements in Integrated Circuit Design
The Role of CMOS Technology in Modern Computing
Complementary Metal-Oxide-Semiconductor (CMOS) technology is the cornerstone of modern computing, underpinning everything from microprocessors to memory chips. The relentless miniaturization of CMOS transistors, guided by Moore’s Law, has led to exponential growth in computing power and efficiency over the past several decades.
The efficiency of CMOS circuits in simulating synapses and neurons, however, is not optimal. It often requires tens of CMOS transistors to emulate a single synapse or neuron, which is inherently inefficient for neuromorphic computing applications. Despite this, CMOS technology has been instrumental in the development of neuromorphic circuits like TrueNorth and Loihi, which aim to mimic the cognitive functions of the human brain.
The following table highlights the contrast between traditional CMOS-based computing and neuromorphic computing in terms of device requirements:
Computing Type | Transistors per Function | Efficiency |
---|---|---|
Traditional CMOS | Multiple | Low for neuromorphic tasks |
Neuromorphic | Single or few | High for synaptic/neuronal simulation |
As we continue to push the boundaries of CMOS technology, the search for more efficient ways to simulate synaptic and neuronal functions is leading to the exploration of alternative materials and device architectures.
Innovations in Memristive and Multi-Terminal Devices
The landscape of semiconductor technology is witnessing a significant shift with the advent of memristive and multi-terminal devices. These innovations are not just theoretical; they are paving the way for more efficient and dense computing architectures. Memristive devices, in particular, have been recognized for their potential in in-memory computing, offering high-density arrays and rapid response times. They are integral to enhancing the performance of artificial neural networks (ANNs) by accelerating multiply–add operations.
However, the simplicity of two-terminal memristive devices comes with a caveat. They typically lack a selective terminal, which can lead to issues such as unselected sneak path currents. This necessitates the use of additional selectors or one-transistor one-memristor (1T1R) structures to ensure proper functionality. On the other hand, multi-terminal devices, including floating-gate transistors, offer improved control over conductance states and are better suited for emulating neural functions.
The integration of these devices into neuromorphic computing systems is a step towards more biologically inspired technology. By using volatile and non-volatile memristors, it is possible to update synaptic weights and perform neuronal integrate–fire functions with greater efficiency. The challenge lies in balancing the trade-off between integration density and the emulation of complex neural behaviors.
Challenges and Solutions in Scaling Down Transistors
As the semiconductor industry continues to push the boundaries of miniaturization, scaling down transistors has become a formidable challenge. The pursuit of smaller transistors encounters physical limitations such as quantum tunneling and leakage currents, which become more pronounced as features shrink. These issues not only affect the reliability of the devices but also their power efficiency.
One of the critical problems faced in this endeavor is the scaling of Static Random-Access Memory (SRAM). SRAM is a cornerstone of modern computing, providing fast access to data. However, as highlighted in the title ‘SRAM Scaling Issues, And What Comes Next,’ the inability of SRAM to scale has led to significant power and performance constraints. To address these challenges, the industry has explored various strategies, including the development of new materials and structures.
For instance, the lateral-gate structure, while offering certain advantages, results in a larger area and reduced integration capability. This necessitates a focus on manufacturing compatibility with existing processes, especially for future oxide-based electrolyte-gated transistors. Moreover, the exploration of highly stable organic/inorganic materials, coupled with advanced encapsulation techniques, is critical for achieving scalability and maintaining device performance below the 5 nm regime.
The table below summarizes some of the key strategies and materials being investigated to overcome the challenges of transistor scaling:
Strategy | Material | Benefit |
---|---|---|
Advanced encapsulation | Organic/Inorganic hybrids | Stability |
New transistor structures | Oxide-based electrolytes | Integration |
3D architecture | Carbon nanotubes | Footprint reduction |
In conclusion, while the challenges of scaling down transistors are significant, ongoing research and innovative solutions continue to drive progress in the field of semiconductor technology.
The Impact of Silicon Synapses on Cognitive Computing
Replicating Human Brain Capabilities
The quest to replicate the human brain’s capabilities in silicon form is a pinnacle challenge in neuromorphic computing. Neuromorphic computers try to replicate that efficiency by forming what are called spiking neural networks, which are essential for achieving brain-like perception abilities with brain-like energy efficiency. The efficiency of the human brain’s intelligence is largely attributed to the dense synaptic interconnections among neurons, a feature that researchers are striving to emulate through neuromorphic electronics.
Neuromorphic integrated circuits, particularly those utilizing CMOS technology, have been developed to mirror the cognitive and energy-efficient capabilities of the human brain. However, the CMOS technology is inherently inefficient for simulating synapses/neurons as it is not specifically designed for this purpose. To create a functional synapse/neuron model in CMOS circuits, multiple CMOS transistors are required. Despite these challenges, notable neuromorphic devices such as TrueNorth and Loihi have emerged, showcasing the potential of this technology.
The implications of successfully replicating a human-like brain in silicon are profound. It could lead to computational entities capable of thinking at speeds millions of times faster than their biological counterparts. This would not only revolutionize our understanding of cognition but also open up new horizons in artificial intelligence, with machines exhibiting reaction times and processing capabilities far beyond human limitations.
Notable Neuromorphic Circuits: TrueNorth and Loihi
The landscape of neuromorphic computing has been significantly shaped by the development of two notable circuits: TrueNorth and Loihi. TrueNorth, developed by IBM, is renowned for its event-driven architecture, which allows for low power operation and massive parallelism. This chip is capable of real-time processing, making it a benchmark in the field of neuromorphic computing.
Intel’s Loihi, on the other hand, represents a leap forward in on-chip learning capabilities. With its manycore processor design, Loihi integrates learning directly into the hardware, paving the way for more adaptive and intelligent computing systems. The recent announcement of Loihi 2 has sparked interest in its potential to further advance open neuromorphic platforms.
Both TrueNorth and Loihi exemplify the strides made in mimicking the efficiency and functionality of the human brain. Their unique architectures and operational paradigms serve as a foundation for future developments in cognitive computing.
Efficiency and Cost Considerations in Synapse/Neuron Simulation
The quest to mimic the human brain’s neural network in silicon has led to significant advancements in neuromorphic computing. The efficiency of neuromorphic devices is paramount, as they strive to replicate the dense synaptic interconnections characteristic of biological brains. These devices, including two-terminal memristive and multi-terminal transistor technologies, offer a more energy-efficient alternative to traditional CMOS circuits for simulating synaptic and neuronal functions.
Cost is another critical factor in the development of neuromorphic circuits. Traditional CMOS technology, while versatile, is not optimized for simulating synapses and neurons, requiring multiple transistors for a single function. This not only increases the complexity but also the cost. In contrast, memristors, with their two-terminal structure, promise ultra-high integration and cost-effectiveness for neuron/synapse simulation. However, challenges such as select issues and sneak path currents must be addressed to realize their full potential.
The following table summarizes the comparison between CMOS and memristive devices in terms of efficiency and cost for synapse/neuron simulation:
Device Type | Efficiency | Cost | Integration Complexity |
---|---|---|---|
CMOS | Low | High | High |
Memristive | High | Low | Low |
As research progresses, the balance between efficiency, cost, and complexity continues to shape the future of neuromorphic computing. The ultimate goal is to achieve brain-like perception abilities with brain-like energy efficiency, a challenge that remains at the forefront of semiconductor technology.
Future Directions in Semiconductor Integrated Circuits
Beyond Traditional CMOS: Exploring New Materials
As the relentless pursuit of miniaturization in semiconductor technology continues, the industry is reaching the physical limits of traditional CMOS scaling. Innovative materials are now pivotal for the next leap in device performance and energy efficiency. Researchers are exploring a variety of new materials, each offering unique advantages over conventional silicon.
Electro-optic polymers, metal-insulator-transition oxides, and 2D materials are being integrated into silicon photonics to achieve ultrafast and ultracompact light modulation. The integration of nonvolatile materials such as charge-trapping materials, ferroelectric materials, and chalcogenide phase change materials (PCMs) is an ongoing trend that promises enhanced device capabilities.
The table below summarizes some of the emerging materials and their potential applications in integrated circuits:
Material Type | Potential Application | Advantages |
---|---|---|
Electro-optic Polymer | Light Modulation | High Speed |
Metal-Insulator-Transition Oxide | Volatile Memory | Low Power Consumption |
2D Materials | Transistors | Flexibility |
Charge-Trapping Materials | Nonvolatile Memory | Enhanced Storage Capacity |
Ferroelectric Materials | Logic Devices | Nonvolatility |
Chalcogenide PCMs | Optical Switches | High Contrast Ratio |
Monolithic integration of these novel materials into existing photonic components is essential for advancing heterogeneous silicon photonic integrated circuits without compromising device reliability.
In-Sensor Processing and Its Implications
The advent of in-sensor processing marks a significant leap in semiconductor technology, enabling a new breed of smart sensors that not only capture data but also process it on the chip. This integration promises to streamline data handling, reduce latency, and enhance the efficiency of devices across various applications.
In-sensor processing leverages the principles of neuromorphic computing, where sensors mimic the biological processes of the human sensory system. By co-integrating sensors with synaptic/neuronal devices, these systems can perform complex tasks such as pattern recognition and perceptual learning, akin to human cognition. For instance, an artificial tactile sensory system can discern nuances in texture and shape, and adapt over time, much like our own tactile system.
The implications of in-sensor processing are far-reaching:
- Reduction in data transmission: By processing data locally, the need for constant communication with central processors is diminished.
- Energy efficiency: Localized processing translates to lower power consumption, which is crucial for battery-operated and remote devices.
- Enhanced privacy: Data can be analyzed and acted upon without leaving the sensor, offering a layer of security against external breaches.
- Real-time responsiveness: Immediate processing allows for quicker reactions in time-sensitive applications, such as autonomous vehicles and healthcare monitoring systems.
Scalability and Integration of Neuromorphic Hardware
The quest for scalability in neuromorphic hardware is a pivotal aspect of its evolution, aiming to replicate the dense synaptic interconnections found in the human brain. As device scaling leads to a smaller footprint and lower power consumption, researchers are pushing the boundaries of current technology to achieve this goal. The channel length of oxide-based transistors, for instance, is showing promise in scaling below the 5 nm regime, thanks to their wide bandgap and low dielectric constant.
Integration of neuromorphic circuits with existing technologies is equally critical. CMOS technology, while not inherently efficient for simulating synapses and neurons, has been adapted to create functional models. This adaptation requires multiple CMOS transistors, highlighting the need for advancements in hardware design. Neuromorphic devices such as TrueNorth and Loihi stand out as notable examples of this integration, showcasing the potential for increased efficiency and scalability in cognitive computing.
To further advance neuromorphic hardware, the following points should be considered:
- Exploration of highly stable organic/inorganic materials for device construction.
- Encapsulation techniques to protect and enhance device longevity.
- Continued research into new ionic neuromorphic functions that can offer more brain-like perception abilities with energy efficiency akin to biological systems.
Conclusion
In summary, the exploration of semiconductor integrated circuits, particularly CMOS technology, has been pivotal in advancing modern technology. As we approach the physical limits of silicon-based ICs, the quest for efficiency and miniaturization has led to the emergence of neuromorphic devices and carbon-based semiconductors. These innovations aim to replicate the human brain’s cognitive abilities and energy efficiency, offering a glimpse into the future of computing. While CMOS technology has been the bedrock of electronics for decades, its inherent limitations in simulating synaptic and neuronal functions necessitate the development of specialized neuromorphic circuits. The integration of memristive devices, ferroelectric transistors, and carbon nanotubes represents the cutting edge of this field, promising to revolutionize how we process information. As we continue to push the boundaries of what is possible, the synergy between biological inspiration and semiconductor technology will undoubtedly unlock new paradigms in computing and beyond.
Frequently Asked Questions
What is the synapse/neuron model in CMOS circuits?
The synapse/neuron model in CMOS circuits attempts to mimic the functionality of biological synapses and neurons using multiple CMOS transistors. Although not inherently efficient for this purpose, CMOS technology has been adapted to create neuromorphic devices that simulate synaptic and neuronal functions.
What are neuromorphic devices and how do they differ from traditional CMOS circuits?
Neuromorphic devices are engineered to emulate the cognitive functions of the human brain, such as learning and memory, by simulating synapses and neurons. Unlike traditional CMOS circuits that are optimized for general computing tasks, neuromorphic devices are specialized for brain-like computational efficiency and include technologies like memristors and multi-terminal transistors.
What is the significance of quantum effects in silicon-based FETs?
As silicon-based integrated circuits reach their physical limits, quantum effects in field-effect transistors (FETs) become more pronounced. These effects can impact the behavior and performance of the transistors at very small scales, which is a significant consideration in the design and operation of modern semiconductor devices.
Why are carbon-based semiconductors considered disruptive in the post-Moore era?
Carbon-based semiconductors, such as carbon nanotubes (CNTs), offer superior electrical transport properties compared to silicon at the same technology nodes. This makes them a promising alternative for further advancements in semiconductor technology as the industry moves beyond the scaling predicted by Moore’s Law.
How do ionic dynamics in electronic materials contribute to neuromorphic computing?
Ionic dynamics in electronic materials, such as those found in oxide-based synaptic transistors, allow for the emulation of biological processes within neuromorphic computing systems. These dynamics enable the devices to perform functions like signal processing and learning, similar to how ions play a role in biological neural computation.
What are some notable neuromorphic circuits and their significance?
Notable neuromorphic circuits include TrueNorth and Loihi, which are designed to replicate the energy-efficient cognitive capabilities of the human brain. These circuits are significant because they represent a departure from traditional computing architectures, focusing instead on brain-inspired processing that could revolutionize how computers handle complex tasks.