Beyond the Basics: Advanced Approaches to Semiconductor Testing
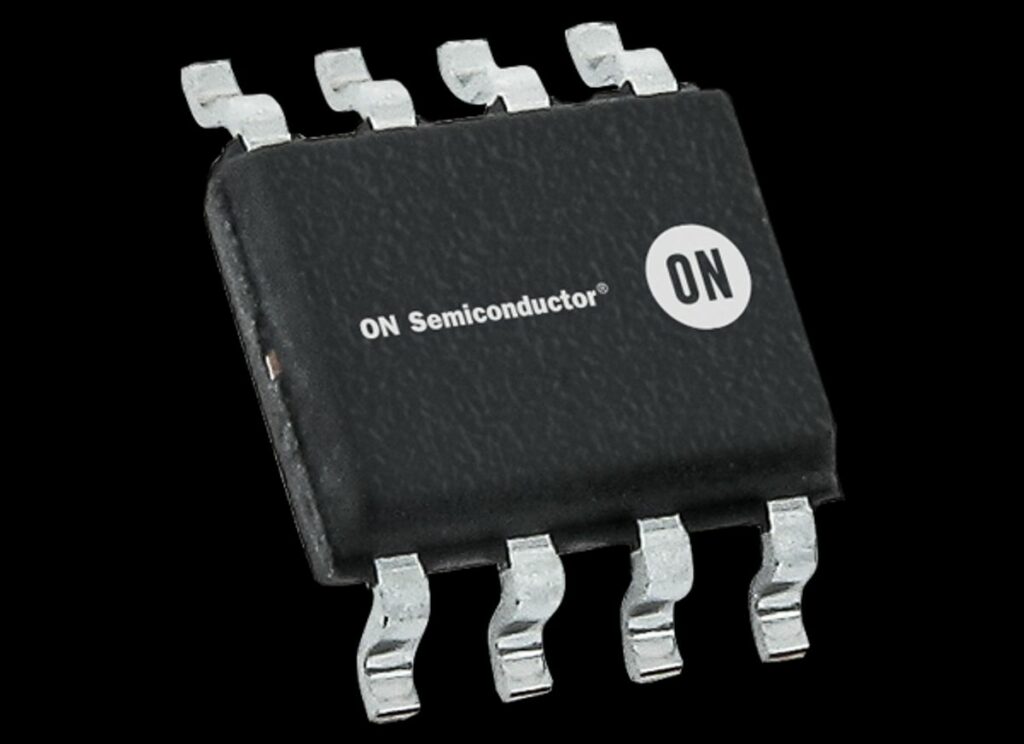
Semiconductor testing is a critical component of the manufacturing process, ensuring the functionality and reliability of devices that power our modern world. As technologies advance, so too must the methods used to test them. This article delves into the sophisticated techniques and strategies currently being deployed and developed to push the boundaries of semiconductor testing further than ever before.
Key Takeaways
- Advanced semiconductor testing strategies are essential for detecting unknown defects and ensuring device reliability in increasingly complex semiconductor architectures.
- Innovative defect inspection techniques and data management systems are being developed to handle the challenges presented by advanced nodes and the vast amount of data generated during testing.
- Standards and benchmarking play a pivotal role in maintaining quality and reliability in semiconductor testing, with entities like NIST providing guidance and oversight.
- Optimization of semiconductor testing is increasingly data-driven, leveraging massive datasets to enhance yield, performance, and manufacturing processes.
- Emerging technologies such as nanoimprint lithography, silicon carbide semiconductors, and advanced packaging are reshaping the testing landscape, necessitating new testing methods and standards.
Innovative Strategies for Unknown Defect Detection
Adapting Models for Customer-Specific Challenges
In the realm of semiconductor testing, the adaptation of AI/ML models to customer-specific challenges is a pivotal step towards achieving high precision and reliability. As noted by industry experts, the key lies in preparing and accessing the necessary data to train robust machine learning models without succumbing to overfitting for particular scenarios. This preparation is a complex task, often involving the collection of vast amounts of data and the careful calibration of models to ensure they remain adaptable and accurate.
The integration of AI/ML models into manufacturing systems must be approached with caution to prevent the occurrence of false positives or escapes. Predictive models that can swiftly adapt to changes are crucial for maintaining smooth operations amidst the intricate complexities of manufacturing. However, manufacturers remain cautious, thoroughly testing models within known parameters before full integration. This cautious approach is essential for establishing trust in the models’ ability to flag genuine issues without causing unnecessary disruptions.
To leverage AI/ML effectively, it is imperative to consider the interactions between different process steps and the influence of design DNA from product tape-outs. This perspective can lead to the development of more generic and scalable models. A shift from isolated innovation to coordinated advancement, from ‘my data’ to ‘our insights’, could significantly enhance yields, process optimization, and defect reduction throughout the supply chain. Standardizing the environment for machine learning models and establishing industry standards could facilitate the selection of the most effective models based on data distribution and decision-making thresholds.
Data Management from Acquisition to Analysis
In the realm of semiconductor testing, data management is a cornerstone that bridges the gap between raw data acquisition and insightful analysis. A fab must not only collect extensive volumes of data but also maintain a robust system of traceability. This ensures the data’s provenance and quality are preserved throughout the semiconductor’s lifecycle. The integration of data from the entire supply chain is pivotal for predicting final test yields, a feat that remains a challenge for many in the industry.
The deluge of data in fabs today necessitates the use of AI/ML solutions to manage petabytes of information. These technologies are crucial for extracting actionable insights, yet their full potential can only be harnessed through rigorous analysis and rapid interpretation. Overcoming hurdles such as ensuring data readiness and accessibility is essential for the successful application of machine learning models.
To illustrate the complexity of data management in semiconductor testing, consider the following table outlining key aspects of the process:
Step | Description |
---|---|
Data Collection | Gathering vast amounts of data from various manufacturing processes. |
Data Traceability | Establishing a system to track data provenance and quality. |
Data Integration | Combining data from different stages of the supply chain. |
Analysis & Action | Applying AI/ML for rapid interpretation and decisive operational adjustments. |
By addressing these steps, manufacturers can enhance their defect detection capabilities, allowing for the identification of issues that may be missed by simpler systems. This multivariate approach provides a more nuanced view of potential failures, leading to process improvements and operational efficiencies.
Leveraging Emerging Patterns for Process Optimization
The semiconductor industry is on the cusp of a transformative era where the detection of emerging patterns plays a pivotal role in process optimization. Advantest’s Leventhal highlights the "potential to detect emerging patterns and bring about optimization of the overall semiconductor manufacturing processes." This not only enhances the efficiency of existing processes but also paves the way for new innovations in equipment design and operational workflows.
A shift from isolated data to a collaborative insight-driven approach is essential. David emphasizes the importance of creating a "standardized environment" for machine learning models, which can lead to significant improvements in yields and defect reduction. The effectiveness of these models is contingent upon the data and its inherent distribution, underscoring the need for industry standards.
The integration of AI and ML technologies is revolutionizing the way data is analyzed, freeing up engineers and technicians to engage in more creative and strategic tasks. Yu points out the untapped opportunities in AI and ML, particularly in understanding the interplay between different process steps and the impact of design DNA from product tape-outs. This could result in more "generic and scalable models," optimizing the semiconductor manufacturing landscape.
Enhancing Defect Inspection Techniques for Advanced Nodes
Improving Current Frameworks and Methodologies
As the semiconductor industry continues to push the boundaries of miniaturization, defect inspection techniques must evolve to keep pace with the ever-decreasing feature sizes of advanced nodes. Traditional methods are being challenged by the complexities introduced by new materials and 3D structures, necessitating a reevaluation and enhancement of current frameworks and methodologies.
One critical area of focus is the adaptation of Scanning Electron Microscopy (SEM) for advanced defect inspection. As semiconductor patterning dimensions shrink, more advanced SEM image-based techniques are needed to detect subtle defects that could impact device performance. This requires not only improvements in the hardware but also in the software algorithms that analyze the SEM images.
To systematically address these challenges, the industry is adopting a multi-faceted approach:
- Enhancing resolution and sensitivity of inspection tools to detect smaller defects.
- Developing sophisticated image processing algorithms that can distinguish between noise and actual defects.
- Integrating machine learning and AI to predict and identify unknown defect patterns.
- Collaborating across the supply chain to ensure that improvements are aligned with the needs of all stakeholders, from equipment manufacturers to chip designers.
Integration of Small-Footprint Scattering Elements
The integration of small-footprint scattering elements into semiconductor testing is a pivotal advancement for enhancing defect inspection capabilities. These elements are crucial for polarization monitoring, which is increasingly important as devices scale down and the inspection of refined lateral dimensions becomes more challenging. The scattering elements are engineered to be highly sensitive to defects that might otherwise go undetected with traditional inspection methods.
In the context of advanced packaging, the necessity for comprehensive wafer inspection is underscored. The scattering elements play a significant role in addressing metrology issues, ensuring that no defects are overlooked. Their integration into existing frameworks not only improves the resolution of inspection techniques but also contributes to the overall reliability of semiconductor devices.
The table below summarizes the benefits of integrating small-footprint scattering elements in semiconductor testing:
Benefit | Description |
---|---|
Enhanced Sensitivity | Allows detection of sub-wavelength defects. |
Improved Resolution | Facilitates the inspection of refined lateral dimensions. |
Greater Reliability | Increases the accuracy of defect identification. |
Scalability | Adaptable to various device sizes and complexities. |
Benchmarking Advanced Imaging Techniques
The evolution of semiconductor testing has seen a significant shift towards integrating advanced imaging techniques with artificial intelligence (AI) to enhance defect inspection. Chipmakers are combining optical inspection and eBeam review to identify and analyze defects with greater precision. This synergy allows for a more comprehensive defect review process, crucial for maintaining quality in advanced nodes.
Benchmarking these techniques involves comparing various imaging methods to determine their effectiveness in different scenarios. For instance, electron holography and pixelated STEM are assessed for their resolution and contrast capabilities when inspecting semiconductor structures. The table below summarizes the performance metrics of these techniques:
Technique | Resolution | Contrast | Applicability |
---|---|---|---|
Electron Holography | High | Moderate | Complex Structures |
Pixelated STEM | Very High | High | Fine Details |
The integration of AI and machine learning (ML) into imaging processes is not expected to be flawless initially but is designed to enhance defect detection over time. As these technologies mature, they will become more adept at managing the ‘data deluge’ in fab and test operations, leading to improved yield and performance.
Standards and Benchmarking in Semiconductor Testing
Overview of Federal Government Standards Activities
The federal government plays a pivotal role in shaping the landscape of semiconductor standards. Federal agencies, such as the National Institute of Standards and Technology (NIST), are instrumental in recommending standards focus areas and priorities for the semiconductor industry. These recommendations are crucial for maintaining a competitive edge in global markets and ensuring the reliability and quality of microelectronics.
Federal standards activities encompass a broad range of initiatives aimed at benchmarking and improving the semiconductor sector. Entities and experts within the field, including those from NIST, contribute to the development of these standards by providing technical insights and guidance. This collaborative effort ensures that the standards remain relevant and effective in addressing the industry’s evolving challenges.
The following list highlights some of the key focus areas identified by federal standards activities:
- Benchmarking electron holography and pixelated STEM for various semiconductor structures
- Setting standards for the chip industry to enhance reliability and performance
- Addressing supply chain security to mitigate threats to the semiconductor ecosystem
- Promoting the integration of small-footprint scattering elements for advanced nodes
These focus areas are not only critical for current industry needs but also lay the groundwork for future advancements in semiconductor testing and manufacturing.
The Role of NIST in Microelectronics Testing
The National Institute of Standards and Technology (NIST) plays a pivotal role in the microelectronics industry, particularly in the realm of testing. NIST’s involvement is critical to ensuring that semiconductor products meet rigorous quality and reliability standards, which are essential for consumer safety and maintaining a competitive edge in the global market.
NIST’s Research & Development programs are designed to propel the advancement of semiconductor technologies. One such initiative is the CHIPS Research and Development (R&D) programs, which aim to enhance the semiconductor sector. These programs are a testament to NIST’s commitment to fostering innovation and supporting the industry’s growth.
Collaboration between NIST and industry stakeholders is fundamental to the development of new testing standards. By working closely with manufacturers, researchers, and other government agencies, NIST helps to establish benchmarks that are both practical and forward-looking, ensuring that the United States remains at the forefront of semiconductor technology.
Setting Industry Benchmarks for Quality and Reliability
In the semiconductor industry, setting benchmarks for quality and reliability is a dynamic process that evolves with technological advancements. The integration of Artificial Intelligence (AI) and Machine Learning (ML) into testing processes is a testament to this evolution. These technologies are not expected to be flawless initially but are designed to enhance processes over time. Collaborative efforts and education about realistic expectations are crucial for the successful application of AI and ML in this field.
The cautious approach of customers towards AI/ML adoption involves rigorous testing within known parameters before full integration. This is due to the industry’s current tolerance for false failures over the risk of missing defects. As such, the establishment of benchmarks must consider the balance between innovation and the potential for error.
To ensure the reliability of data used in AI/ML applications, a robust system of traceability is essential. This system must guarantee the data’s provenance and quality throughout the semiconductor’s lifecycle. The table below illustrates the key aspects of data management that contribute to setting industry benchmarks:
Aspect | Description |
---|---|
Data Volume | Collection of extensive data volumes from the fab. |
Data Traceability | Establishment of a traceable data system to ensure quality. |
Supply Chain Integration | Integration of data from the entire supply chain for better yield prediction. |
Knowledge Application | Ability to apply knowledge from back end to front end processes. |
The benchmarks set today will not only define current standards but also shape the future of semiconductor testing as we continue to embrace emerging technologies.
The Role of Data in Semiconductor Test Optimization
Managing the Data Deluge in Fab and Test Operations
The modern semiconductor fabrication plant, or fab, is a data-intensive environment where the ability to manage and analyze vast quantities of information is critical to operational success. AI and machine learning (AI/ML) technologies are increasingly seen as vital tools for handling the petabytes of data generated, ensuring that actionable insights can be extracted efficiently and effectively.
However, the integration of AI/ML into fab operations is not without its challenges. Fabs must ensure the quality and relevance of data, cope with the computational demands of processing large datasets in real-time, and navigate the cultural changes necessary to adopt these technologies. This is particularly complex when it involves collaboration between competing companies that may be reluctant to share data.
To illustrate the complexity of data management in fabs, consider the following points:
- Traceability: Establishing a system that maintains the provenance and quality of data throughout the semiconductor lifecycle.
- Integration: Combining data from the entire supply chain to improve yield prediction accuracy.
- Model Deployment: Ensuring diverse data types and adequate production sampling are considered when building predictive models.
- Process Control: Utilizing AI/ML for real-time process adjustments, detecting deviations, and preventing defect trends.
Utilizing Data for Enhanced Yield and Performance
In the quest for superior semiconductor yield and performance, the strategic use of data is paramount. A pivot from isolated innovation to coordinated advancement is crucial, transforming ‘my data’ into ‘our insights’ to unlock improvements in yields and defect reduction. This requires a standardized environment for machine learning models, which are contingent on the data’s inherent distribution.
The effective use of machine learning in enhancing chip yield hinges on the integration of vast data sets. As Dieter Rathei, CEO of DR Yield, emphasizes, the ability to experiment with various AI models is predicated on the availability of comprehensive data. This shift not only facilitates the discovery of new modeling opportunities but also unveils potential enhancements in process, equipment design, and operational workflows.
To ensure the success of AI/ML applications, a fab must not only amass extensive data but also maintain a traceable system to guarantee data quality throughout the semiconductor’s lifecycle. Integrated data from the entire supply chain leads to more accurate predictions of final test yield, allowing for the application of insights from the back end to the front end of the manufacturing process.
Data-Driven Approaches to Semiconductor Manufacturing
In the realm of semiconductor manufacturing, the integration of Artificial Intelligence (AI) and Machine Learning (ML) is revolutionizing the way data is utilized. The ability to harness extensive volumes of data is pivotal for optimizing manufacturing processes. A fab’s success hinges on not just data collection but also on establishing a robust traceability system to ensure data quality and provenance throughout the semiconductor’s lifecycle.
Effective AI implementation requires comprehensive data collection, as incomplete data sets can render even the most advanced algorithms ineffective. The heterogeneous nature of semiconductor manufacturing data, ranging from equipment performance metrics to wafer inspection results, presents a unique challenge in data harmonization.
The collaboration between semiconductor value chain partners, such as foundries and fabless companies, is crucial for the successful application of ML algorithms. However, historical defensive postures can often hinder progress. By leveraging AI/ML, the industry can shift focus from mundane data analysis to strategic planning and creative problem-solving. The table below highlights the key aspects of data-driven approaches in semiconductor manufacturing:
Aspect | Description |
---|---|
Data Collection | Comprehensive and diverse data sets from the entire manufacturing process. |
Data Quality | Systems of traceability to ensure data integrity and provenance. |
AI/ML Integration | Use of algorithms to analyze data and optimize processes. |
Collaboration | Sharing of data between value chain partners to enhance ML algorithm effectiveness. |
Innovation | AI/ML enabling engineers to focus on innovation and strategic tasks. |
Emerging Technologies and Their Impact on Testing
Nanoimprint Lithography and Its Testing Implications
Nanoimprint lithography (NIL) is a promising technology that offers high-resolution patterning capabilities essential for next-generation semiconductor devices. The precision of NIL demands rigorous testing protocols to ensure defect-free patterns and high throughput. The testing implications of NIL are multifaceted, involving both the imprint process and the resulting structures.
Key aspects of NIL testing include the assessment of template fidelity, imprint uniformity, and layer-to-layer alignment. These factors are critical for maintaining the integrity of the nanostructures that NIL creates. Additionally, the mechanical properties of the resist and the release characteristics of the template are vital to achieving consistent imprints.
To illustrate the complexity of NIL testing, consider the following table outlining common testing parameters and their typical targets:
Parameter | Target |
---|---|
Line Edge Roughness (LER) | < 3 nm |
Line Width Variation (LWV) | < 5 nm |
Overlay Accuracy | < 5 nm |
As the industry moves towards smaller feature sizes and more complex device architectures, the role of NIL in semiconductor manufacturing is set to expand. With this expansion comes the need for enhanced testing methods that can keep pace with the technology’s advancements. The integration of intelligent manufacturing solutions, as discussed in the white paper ‘Unlocking Value: The Power of AI in Semiconductor Test’, could be pivotal in addressing these challenges.
The Advent of Silicon Carbide and Power Semiconductor Testing
The introduction of Silicon Carbide (SiC) semiconductors marks a significant shift in power electronics. Known for their robustness, SiC devices are increasingly favored for their ability to handle high voltages and temperatures, making them ideal for power applications. This has necessitated the development of specialized testing protocols to ensure device reliability under extreme conditions.
Testing for SiC semiconductors involves a series of steps to evaluate their performance and durability. These include high-temperature operating life (HTOL) tests, which assess long-term reliability, and specialized gate-oxide integrity tests to measure resistance to voltage stress. Additionally, dynamic testing is crucial for understanding the switching characteristics and loss profiles of SiC devices in real-world scenarios.
As the industry continues to innovate, the testing landscape for SiC and power semiconductors will evolve. Collaborative efforts between manufacturers and testing facilities are essential to establish benchmarks that reflect the unique properties of these advanced materials.
Evaluating the Testing Needs for Chiplets and Advanced Packaging
The integration of chiplets into advanced packaging architectures has introduced new complexities in semiconductor testing. Testing semiconductor dies is crucial for enhancing product reliability and reducing costs. Design for Testability (DFT) techniques are pivotal in addressing these challenges, with methods such as At-speed Scan playing a significant role.
As the industry moves towards more modular designs, the testing needs for chiplets and advanced packaging must be re-evaluated. The following points highlight key considerations:
- Ensuring compatibility and interoperability between chiplets from different vendors.
- Developing comprehensive test strategies that encompass the entire system, not just individual dies.
- Adapting test equipment and methodologies to handle the increased density and complexity of connections.
The shift towards chiplet-based systems is not only a technical evolution but also a business one. It necessitates a strategic approach to testing that aligns with the overarching goals of system performance and cost-effectiveness.
Conclusion
In the intricate dance of semiconductor testing, we have ventured beyond the basics to explore advanced approaches that cater to the ever-evolving landscape of microelectronics. This article has underscored the importance of hunting the unknown, adapting to unique customer needs, and leveraging emerging patterns for optimization. As we’ve seen, the journey of semiconductor testing is not a solitary one; it is a collaborative effort that involves knowledge centers, technical papers, and industry experts who continuously push the boundaries of what’s possible. The insights from entities like Advantest and standards from NIST, along with the contributions from thought leaders across the field, pave the way for innovation and excellence in semiconductor manufacturing. As we embrace these advanced approaches, we stand on the cusp of a new era where the precision and efficiency of semiconductor testing are paramount to the technological advancements that drive our world forward.
Frequently Asked Questions
What are innovative strategies for unknown defect detection in semiconductors?
Innovative strategies include adapting models for customer-specific challenges, managing data from acquisition to analysis, and leveraging emerging patterns to optimize semiconductor manufacturing processes.
How are defect inspection techniques enhanced for advanced semiconductor nodes?
Enhancement involves improving current frameworks and methodologies, integrating small-footprint scattering elements for better monitoring, and benchmarking against advanced imaging techniques.
What role do federal standards play in semiconductor testing?
Federal government standards, particularly those set by NIST, play a crucial role in establishing benchmarks for quality and reliability in semiconductor testing.
How is data utilized in optimizing semiconductor test operations?
Data is managed to handle the volume in fab and test operations, used to enhance yield and performance, and applied in data-driven approaches to manufacturing.
What emerging technologies are impacting semiconductor testing?
Technologies such as nanoimprint lithography, silicon carbide semiconductors, and the development of chiplets and advanced packaging are changing the landscape of semiconductor testing.
Why is tackling unknown defects as important as perfecting known issues in semiconductor testing?
Identifying and addressing unknown defects is critical to ensure the adaptability of solutions to specific customer needs and to maintain the integrity and advancement of semiconductor manufacturing processes.