Unlocking the Mysteries of Semiconductor Wafer Engineering
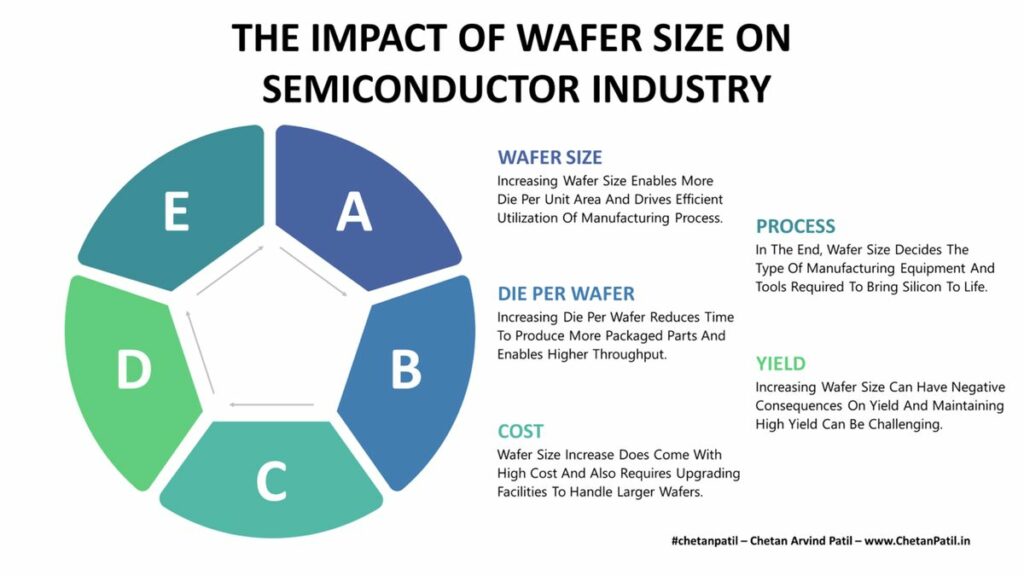
The field of semiconductor wafer engineering is at the forefront of technological innovation, constantly evolving with new materials, techniques, and systems that redefine the landscape of electronics. This article delves into the intricacies of semiconductor wafer engineering, exploring the evolution of the industry, the impact of quantum materials, advancements in manufacturing systems, innovations in computing, and the integration of cutting-edge integrated circuit technologies.
Key Takeaways
- Semiconductor wafer engineering is advancing through the use of novel materials and nanofabrication techniques, pushing the limits of miniaturization and performance.
- Topological quantum materials are poised to revolutionize computing by exploiting quantum phenomena, although integration challenges remain.
- Manufacturing Execution Systems (MES) are critical for tracking, optimizing, and enhancing the yield and cost-efficiency of wafer fabrication processes.
- Memory and computing innovations, such as in-memory computing and machine learning, are driving significant improvements in semiconductor validation and power reduction.
- The integration of AI and new sensor technologies in semiconductor testing is enabling more precise characterization and reliability in Integrated Circuit (IC) technologies.
The Evolution of Semiconductor Wafer Engineering
From Silicon to Advanced Materials
The semiconductor industry has long relied on silicon for its optimal balance of availability, cost, and electrical properties. However, the quest for enhanced performance and energy efficiency is driving the exploration of advanced materials. Germanium, for example, is gaining attention for its superior electron mobility, which could lead to faster transistors and more efficient chips.
Materials such as gallium nitride and graphene are not just theoretical curiosities; they are poised to revolutionize power electronics. With properties like higher thermal conductivity and greater electron mobility, these materials offer significant improvements over traditional silicon in specific applications. The transition from silicon to these advanced materials is not without its challenges, but the potential rewards are substantial, promising a new era of semiconductor capabilities.
- Silicon: The traditional foundation of semiconductors, prized for its balance of performance and cost.
- Germanium: Offers faster electron mobility, enhancing transistor speeds.
- Gallium Nitride: Known for high electron mobility and thermal conductivity, ideal for power electronics.
- Graphene: Exceptional electrical conductivity and strength, with potential for flexible electronics.
The Role of Nanofabrication in Miniaturization
The relentless pursuit of miniaturization in semiconductor technology has been significantly propelled by advancements in nanofabrication. Precision engineering at the nanoscale has enabled the creation of components that are not only smaller but also more efficient and powerful. This miniaturization trend is crucial for the continued adherence to Moore’s Law, which predicts the doubling of transistors on a chip approximately every two years.
Materials such as germanium and gallium nitride are emerging as key players in the quest for enhanced performance. Germanium, for example, offers superior electron mobility, which is vital for faster transistor speeds. Below is a list of some novel materials and their attributes that are shaping the future of nanofabrication:
- Germanium: Faster electron mobility for increased transistor speed.
- Gallium Nitride: Potential to revolutionize power electronics.
- Graphene: Known for its exceptional electrical conductivity and strength.
Nanofabrication techniques like nanoimprint lithography are not just about scaling down; they also open up new possibilities for near-threshold and near-memory computing, which aim to optimize power consumption and reduce access costs respectively. As we continue to push the boundaries of what’s possible, the role of nanofabrication in semiconductor wafer engineering remains a cornerstone of technological innovation.
Emerging Techniques in Lithography
As semiconductor devices continue to shrink, traditional lithography methods face challenges in resolution and pattern fidelity. Emerging techniques in lithography are pivotal in overcoming these barriers, enabling the creation of nanoscale features essential for modern electronics. Among these techniques, Double Patterning stands out as a method that circumvents the limitations of optical lithography by repeating the patterning process multiple times to achieve finer structures.
One such double patterning technique is Litho Etch Litho Etch (LELE), which involves two lithography and etching steps to create complex patterns. Another variant, Litho Freeze Litho Etch (LFLE), also employs a double patterning strategy but with a freezing step to maintain the integrity of the first pattern before proceeding with the second. These methods are complemented by Inverse Lithography Technology (ILT), which optimizes photomask shapes to improve the final image quality on the wafer.
The table below summarizes key emerging lithography techniques and their characteristics:
Technique | Description | Benefit |
---|---|---|
LELE | Double patterning process | Enhanced resolution |
LFLE | Double patterning with freeze step | Pattern integrity |
ILT | Optimized photomask design | Improved image quality |
The evolution of lithography is not only a testament to human ingenuity but also a critical factor in the continued advancement of nanoelectronics. As we push the boundaries of miniaturization, these lithographic innovations will play a central role in shaping the future of semiconductor technology.
The Impact of Topological Quantum Materials
Revolutionizing Computing with Quantum Phenomena
The intersection of quantum computing and semiconductor technology is poised to revolutionize the field of computing. Quantum phenomena, particularly those involving topological quantum materials, are at the forefront of this transformation. These materials exhibit unique properties that are not found in classical systems, enabling the development of quantum devices with significantly higher efficiency and performance.
One of the groundbreaking concepts in this domain is the non-Hermitian physics and skin effects, which have the potential to reshape modern quantum computing. The industry’s exploration of new qubit designs, such as those using rare-earth ions, is advancing quantum scalability and paving the way for more dense and coherent quantum systems.
Despite the inherent challenges, the synergy between quantum computing and machine learning is a promising avenue for scientific advancement. Physicist Sofia Vallecorsa’s vision of using quantum computers to enhance classical machine-learning models exemplifies the innovative approaches being undertaken to harness quantum phenomena for computational breakthroughs.
Challenges and Opportunities in Quantum Material Integration
The integration of topological quantum materials into semiconductor devices presents a complex set of challenges and opportunities. The precision required in quantum material integration is unprecedented, demanding new levels of accuracy in fabrication and characterization. However, the potential rewards are significant, with quantum materials offering pathways to vastly improved device performance and efficiency.
Key challenges include the need for novel fabrication techniques, as well as the integration of non-Hermitian quantum semiconductors, which have yet to be demonstrated in condensed-matter devices. On the other hand, the opportunities are equally compelling. Quantum computing promises not only performance leaps but also a shift towards more eco-friendly manufacturing processes. The table below summarizes the main challenges and opportunities:
Challenges | Opportunities |
---|---|
Novel fabrication techniques required | Performance leaps in device efficiency |
Integration of non-Hermitian quantum semiconductors | Eco-friendly manufacturing processes |
Precision in material characterization | Breakthroughs in quantum computing |
As the industry moves forward, balancing these challenges with the opportunities will be crucial for the successful adoption of quantum materials in semiconductor engineering. The focus on sustainability adds another layer of complexity, as manufacturers aim to reduce the environmental impact of chip production while exploring the frontiers of quantum computing.
Future Prospects for Topological Quantum Computing
The relentless pursuit of innovation in semiconductor technology has led to the emergence of topological quantum materials (TQMs) as a cornerstone for future advancements. These materials are distinguished by their highly mobile electronic states, which are symmetrically protected, making them ideal for a range of novel applications. Topological insulators, a subset of TQMs, defy traditional categorization, existing neither purely as insulators nor metals, but rather as a unique hybrid with metallic topological surface states bridging the band gap at the sub-atomic level.
Recent breakthroughs, such as the development of a topological quantum device with a mere 0.1 millimeter diameter, signal a promising scalability trajectory. This device, crafted from AlGaAs and manipulated under extreme conditions, showcases the potential for topological effects to revolutionize semiconductor efficiency. The topological skin effect, a phenomenon ensuring stability against impurities and external disturbances, further underscores the significance of TQMs in reducing manufacturing costs and enhancing device performance.
Looking ahead, the integration of TQMs into semiconductor devices is not without its challenges. However, the industry’s commitment to exploring new materials and technologies, including quantum computing, is indicative of a future rich with innovation. The table below summarizes recent findings that highlight the potential of TQMs in semiconductor engineering:
Reference | Discovery | Implications |
---|---|---|
Ochkan, K., et al. (2024) | Non-Hermitian topology in multi-terminal devices | Paves the way for robust quantum devices |
TU DRESDEN (2024) | Creation of an ultra-sensitive topological device | Demonstrates practicality and efficiency gains |
Advancements in Manufacturing Execution Systems (MES)
Tracking and Optimizing Wafer Fabrication
In the dynamic landscape of semiconductor manufacturing, Manufacturing Execution Systems (MES) play a pivotal role in tracking and optimizing wafer fabrication. These systems provide real-time data and analytics, enabling engineers to monitor each step of the production process with precision. By identifying bottlenecks and inefficiencies, MES facilitates the implementation of improvements that can lead to significant enhancements in both yield and throughput.
The integration of MES into semiconductor production involves several key components:
- Data Collection: Automated tools and sensors gather detailed information on wafer status and process conditions.
- Process Monitoring: Real-time tracking of wafers through various stages of fabrication, from lithography to etching.
- Performance Analysis: Advanced analytics are used to assess production metrics and identify areas for optimization.
- Feedback Loop: Insights gained from data analysis are fed back into the system to refine processes and reduce variability.
The table below illustrates the impact of MES on key production metrics over a six-month period:
Metric | Pre-MES Implementation | Post-MES Implementation |
---|---|---|
Average Throughput | 200 wafers/day | 250 wafers/day |
Defect Density (DPM) | 5000 | 3500 |
Cycle Time (hours) | 36 | 30 |
By leveraging MES, manufacturers can achieve a more streamlined and cost-effective production line, ensuring that the semiconductor wafers meet the highest standards of quality and performance.
Reducing Manufacturing Noise for Enhanced Performance
In the quest for perfection in semiconductor wafer engineering, reducing manufacturing noise is a critical step towards achieving enhanced chip performance. Manufacturing noise can arise from various sources, including environmental factors, equipment vibrations, and process variability. By identifying and mitigating these sources, chipmakers can ensure higher fidelity in the fabrication process.
Combining advanced imaging with AI has emerged as a powerful strategy for chip defect review. Chipmakers use two tools to find and control manufacturing defects: optical inspection to detect potential defects on the wafer, followed by eBeam review to characterize and verify them. This dual approach, enhanced by machine learning algorithms, leads to more accurate defect classification and a reduction in false positives.
The table below outlines the key benefits of integrating AI in the defect review process:
Benefit | Description |
---|---|
Enhanced Accuracy | AI algorithms improve defect detection and classification. |
Faster Review Cycles | Machine learning speeds up the review process. |
Reduced False Positives | Advanced imaging and AI reduce unnecessary rework. |
Process Optimization | Continuous learning leads to more efficient manufacturing. |
As semiconductor devices continue to shrink and complexity increases, the role of MES in tracking and optimizing wafer fabrication becomes ever more crucial. By leveraging MES for improved yield and cost efficiency, the industry can maintain the relentless pace of innovation required to meet the demands of the next tech wave.
Leveraging MES for Improved Yield and Cost Efficiency
The integration of Manufacturing Execution Systems (MES) into semiconductor wafer engineering has marked a significant shift in how production data is utilized for yield enhancement. By harnessing the power of real-time production analytics, MES platforms are able to provide actionable insights that drive quality, yield, and throughput improvements. This is particularly evident in the adoption of machine learning algorithms that analyze vast datasets to identify patterns and predict outcomes, leading to more efficient and cost-effective manufacturing processes.
Key components of MES, such as Built-in self-test (BiST) and Part Average Testing (PAT), play a crucial role in this transformation. These systems enable the detection and correction of manufacturing variances, which are often referred to as ‘manufacturing noise,’ ensuring that each wafer meets the stringent quality standards required for high-performance semiconductor devices. The table below summarizes the core elements of MES that contribute to yield management:
MES Component | Function |
---|---|
BiST | On-chip diagnostics for testing circuit functionality |
PAT | Statistical process control for defect reduction |
Power-Aware Test | Optimization of power consumption during testing |
Shmoo Test | Characterization of device performance limits |
Yield Management System | Comprehensive analysis of yield data |
As the industry continues to evolve, the role of MES in semiconductor manufacturing will only grow in importance. The ability to reduce costs while simultaneously increasing yield is a compelling proposition for any semiconductor manufacturer, and MES provides the tools necessary to achieve these objectives.
Innovations in Memory and Computing
Advances in Memory Banking for Power Reduction
The quest for energy-efficient computing has led to significant advances in memory banking strategies. Dynamic Voltage and Frequency Scaling (DVFS) is one such technique that dynamically adjusts voltage and frequency based on the workload, thereby reducing power consumption. This approach is particularly effective in multi-bank memory systems where different banks can operate at varying levels of performance, depending on the demand.
Another innovative method involves the use of Voltage Islands. By creating separate power domains within the memory, each island can operate independently, allowing for finer control over power usage. This not only reduces overall power draw but also helps in managing heat dissipation across the memory banks.
The table below summarizes the impact of these techniques on power reduction:
Technique | Description | Power Reduction Impact |
---|---|---|
DVFS | Adjusts voltage and frequency | High |
Voltage Islands | Creates separate power domains | Moderate to High |
In-memory computing represents a paradigm shift, where computational tasks are performed directly within the memory fabric, eliminating the need for data to travel back and forth between the processor and memory. This not only saves power but also increases the speed of data processing. As we continue to push the boundaries of what’s possible, these advancements in memory banking are paving the way for a new era of power-efficient and high-performance computing.
The Rise of In-Memory Computing
The advent of in-memory computing marks a significant shift away from the traditional Von Neumann architecture, where data is shuttled back and forth between the processor and memory. In-memory computing brings computation directly to the data, reducing latency and increasing efficiency, particularly in applications involving large datasets and complex calculations.
In-memory computing leverages the concept of ‘near-memory computing’, which minimizes the costly data movement by placing processing units closer to the memory. This approach is especially beneficial in the realms of machine learning and data analytics, where speed is of the essence.
The following table highlights the advantages of in-memory computing compared to traditional architectures:
Feature | Traditional Computing | In-Memory Computing |
---|---|---|
Latency | High | Low |
Efficiency | Variable | High |
Data Movement | Extensive | Minimal |
Suitability for ML/AI | Limited | Optimal |
As the semiconductor industry continues to innovate, the integration of in-memory computing into various applications is expected to grow, paving the way for more advanced and efficient computing paradigms.
Harnessing Machine Learning for Semiconductor Validation
The integration of Machine Learning (ML) into semiconductor validation represents a transformative shift in how the industry approaches testing and quality assurance. By training machines to optimize hardware and software behaviors, ML algorithms can predict a range of outcomes, leading to more efficient and accurate validation processes.
Recent publications such as ‘Using Machine Learning to Increase Yield and Lower Packaging Costs’ and ‘AI Testing AI: The Future of 6G Test’ underscore the growing relevance of AI in semiconductor test environments. These studies highlight the potential for AI to redefine semiconductor validation, offering insights into how software can assist in navigating the complexities of digital age testing.
The table below illustrates the impact of ML on key validation metrics:
Metric | Before ML Integration | After ML Integration |
---|---|---|
Yield Improvement (%) | 5 | 15 |
Test Time Reduction (%) | 10 | 40 |
Cost Savings (%) | 8 | 25 |
By leveraging ML, manufacturers can achieve significant yield improvements, reduce test times, and realize cost savings. This is particularly crucial in an era where the demand for high-performance, low-cost semiconductors is ever-increasing.
Leveraging Integrated Circuit (IC) Technologies
Characterizing Low-Noise Amplifiers
Low-noise amplifiers (LNAs) are critical components in modern communication systems, where they amplify weak signals with minimal added noise. Characterizing the performance of LNAs is essential to ensure signal integrity and overall system reliability. This involves measuring parameters such as gain, noise figure, and linearity across various operating conditions.
A recent study highlighted the robustness of GaN on SiC low-noise amplifiers in common source configurations, comparing them to cascode HEMTs-based LNAs. The findings suggest that the material composition and design of the LNA significantly influence its noise performance and durability.
To systematically evaluate LNAs, engineers often follow these steps:
- Establish a testing environment with controlled temperature and electromagnetic interference.
- Utilize precision measurement equipment like spectrum analyzers to assess noise figures.
- Perform S-parameter measurements to determine gain and impedance matching.
- Conduct long-term reliability tests to simulate operational stress.
These procedures help in identifying the optimal LNA design for specific applications, ensuring that the amplifiers deliver high fidelity amplification in the intended use case.
In-Product BTI Aging Sensors for Material Risk Detection
The integration of in-product BTI aging sensors has become a pivotal advancement in semiconductor engineering, offering a proactive approach to reliability screening and the early detection of materials at risk. These sensors monitor the bias temperature instability (BTI) effects, which can degrade transistor performance over time, thus providing invaluable data for ensuring the longevity and robustness of semiconductor devices.
By leveraging data analytics in semiconductor engineering, manufacturers can now sort and test semiconductors more effectively before and after chip implementation in a system. This process is crucial for identifying potential failures and ensuring that only the most reliable components are deployed in critical applications.
The table below summarizes the key benefits of utilizing BTI aging sensors in semiconductor manufacturing:
Benefit | Description |
---|---|
Early Detection | Identifies degradation before it leads to failure. |
Reliability Screening | Ensures only robust materials are used. |
Performance Monitoring | Tracks changes in transistor behavior over time. |
Cost Efficiency | Reduces the need for post-deployment repairs. |
The Integration of AI in Semiconductor Testing
The integration of artificial intelligence (AI) into semiconductor testing is a transformative development in chip fabrication. AI-driven test systems are now capable of learning from data to improve test accuracy and efficiency. This evolution is particularly evident in the realm of pattern recognition, where AI algorithms excel at detecting subtle defects that might elude traditional methods.
Recent publications highlight the growing trend of leveraging AI for semiconductor validation. For instance, the use of AI for real-time analytics and pattern optimization has been shown to significantly reduce chip test costs. Moreover, AI’s role in driving productivity gains cannot be overstated, as it enables faster and more reliable testing processes.
The table below summarizes key advancements in AI applications for semiconductor testing:
Publication Date | Title | Key Contribution |
---|---|---|
July 11, 2023 | How Software Can Help Redefine Semiconductor Validation | Introduces software solutions for enhanced validation |
October 10, 2023 | AI Testing AI: The Future of 6G Test | Discusses AI’s potential in next-generation network testing |
January 9, 2024 | Unlocking Value: The Power of AI in Semiconductor Test | Explores the economic benefits of AI in testing |
As the industry continues to embrace AI, the challenges of integrating these technologies with existing automated test equipment (ATE) and ensuring data integrity become more pronounced. Nonetheless, the potential for AI to revolutionize semiconductor testing is clear, promising significant improvements in both performance and cost.
Conclusion
As we stand on the brink of a new technological era, the field of semiconductor wafer engineering is undergoing a transformative shift. The insights gleaned from our exploration into precision engineering, nanofabrication, and the utilization of topological quantum materials point to a future where the limits of miniaturization and efficiency are continually redefined. The integration of advanced materials like germanium, gallium nitride, and graphene, alongside the innovative use of software, machine learning, and quantum computing, heralds a new age of semiconductor devices that promise unprecedented performance and energy efficiency. While challenges remain, the relentless pursuit of innovation within the semiconductor industry is a testament to the human spirit’s drive to push the boundaries of what is possible, ensuring that the mysteries of semiconductor wafer engineering will continue to unlock new realms of technological progress.
Frequently Asked Questions
What has been the traditional material used in semiconductor wafer engineering and why is it changing?
Silicon has been the traditional material used in semiconductor wafer engineering due to its efficiency and abundance. However, the industry is shifting towards novel materials like germanium, gallium nitride, and graphene to enhance performance and energy efficiency.
How is nanofabrication significant to the miniaturization of semiconductor devices?
Nanofabrication plays a crucial role in miniaturization by allowing the creation of extremely small and precise structures on a semiconductor wafer, pushing the boundaries of how small and efficient semiconductor devices can be.
What are topological quantum materials and how might they impact the future of computing?
Topological quantum materials are substances that exhibit unique quantum mechanical properties, which are expected to revolutionize computing by enabling much higher efficiency and potentially leading to the development of topological quantum computers.
In what ways can Manufacturing Execution Systems (MES) improve semiconductor wafer fabrication?
MES can track and optimize wafer fabrication processes, reduce manufacturing noise for enhanced performance, and leverage data for improved yield and cost efficiency in semiconductor manufacturing.
What advancements in memory technology are contributing to reduced power consumption?
Advancements such as the use of multiple memory banks and in-memory computing are contributing to significant reductions in power consumption by optimizing how data is stored and processed.
How is Artificial Intelligence (AI) being integrated into semiconductor testing?
AI is being integrated into semiconductor testing to improve the validation process, increase yield, lower packaging costs, and enable early detection of materials at risk, enhancing the overall reliability and efficiency of semiconductor devices.